![The 4 Major Corporate Fears About AI [and How Arkane Crushes Them]](https://arkanecloud.com/wp-content/uploads/elementor/thumbs/image-fears-r83x02fmwkg0873grkx2f5jvdsy2k1b00h5jqz1vi0.png)
AI Training and Inference Capabilities of the Nvidia A100
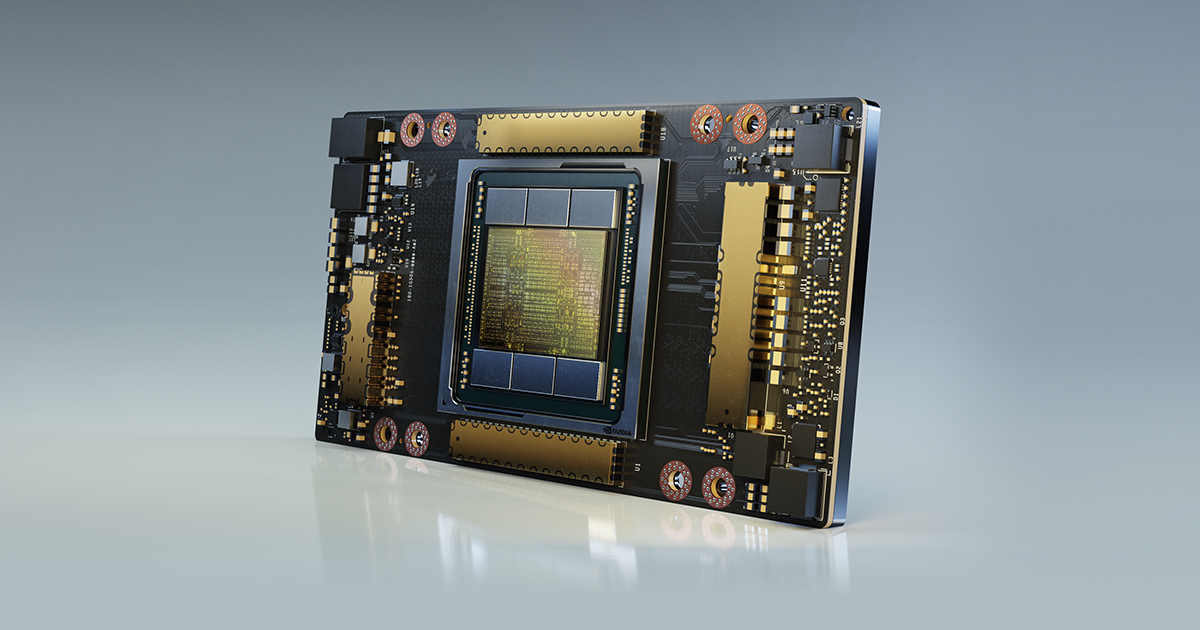
Overview of the Nvidia A100 Architecture
A Revolutionary Leap in GPU Technology: The Ampere Architecture
The Nvidia A100, based on the groundbreaking Ampere architecture, represents a significant leap forward in GPU technology. This architecture is a successor to both the Volta and Turing architectures, embodying a fusion of advances from both. The A100, introduced in May 2020, is crafted with a staggering 54 billion transistors, making it the largest 7 nanometer chip ever built at that time. It showcases a blend of sheer computing power and energy efficiency, a crucial factor in modern AI and HPC (High-Performance Computing) applications. This advanced GPU architecture is designed to address some of the most demanding challenges in scientific, industrial, and business realms by accelerating AI and HPC workloads at unprecedented scales.
The Ampere GPU: A Closer Look at the A100
The A100 accelerator is a testament to Nvidia’s engineering prowess, featuring 19.5 teraflops of FP32 (single-precision floating-point) performance, 6912 CUDA cores, and a generous 40GB of graphics memory. This configuration is coupled with a remarkable 1.6TB/s of graphics memory bandwidth, enabling the A100 to handle massive and complex data sets efficiently. Notably, the A100 was initially launched as a part of the third generation of the DGX server, which included eight A100 GPUs, showcasing the potential for scalable, high-performance AI infrastructure.
Innovations in Tensor Cores: Elevating AI and HPC Performance
The third-generation Tensor Cores in the A100 mark a significant evolution from their predecessors in the Volta architecture. These Tensor Cores are engineered to deliver dramatic speedups in AI, reducing training times from weeks to hours and providing substantial acceleration to inference processes. The introduction of new precisions like Tensor Float 32 (TF32) and floating point 64 (FP64) in the Ampere architecture expands the capabilities of the Tensor Cores, making them more versatile for AI training and inference. TF32, in particular, operates like FP32 but offers speedups of up to 20X for AI applications without any code changes. Additionally, the A100’s support for bfloat16, INT8, and INT4 data types further extends its versatility, making it an adaptable accelerator for a wide array of AI workloads.
Scaling New Heights with the A100
The A100’s design allows it to seamlessly integrate into the fabric of data centers, powering a wide range of AI and HPC applications. Its ability to scale to thousands of GPUs using technologies like NVLink, NVSwitch, PCI Gen4, and NVIDIA InfiniBand, and the NVIDIA Magnum IO SDK, empowers researchers to deliver real-world results rapidly and deploy solutions at scale. This scalability is pivotal in tackling some of the most complex AI challenges, such as training conversational AI models or deep learning recommendation models (DLRM), where the A100 demonstrates up to a 3X throughput increase compared to its predecessors.
In summary, the Nvidia A100, with its advanced Ampere architecture, represents a monumental step in GPU technology, offering unprecedented performance and scalability for AI and HPC applications. Its innovative design and powerful Tensor Cores make it an indispensable tool for researchers and enterprises looking to harness the full potential of AI and data analytics.
Enhancing AI Training and Inference
The Pinnacle of AI Performance: NVIDIA A100 in Action
The NVIDIA A100, leveraging the innovative Ampere architecture, is at the forefront of accelerating AI training and inference, offering a generational leap in performance. With the A100, NVIDIA has achieved a 20x improvement in computing performance over its predecessors, a feat that dramatically enhances the efficiency of AI workloads. This leap in performance is not just theoretical but is being actively realized in various applications, from cloud data centers to scientific computing and genomics. The A100’s capabilities extend to diverse and unpredictable workloads, making it a versatile tool for scaling up AI training and scaling out inference applications, including real-time conversational AI.
NVIDIA DGX A100: A New Era of Supercomputing
At the heart of this revolution is the NVIDIA DGX A100 system, a supercomputing AI infrastructure that integrates eight A100 GPUs, offering up to 5 petaflops of AI performance. This system is designed to handle the most demanding AI datasets, making it an essential building block for AI data centers. The DGX A100 system is not just a powerhouse in performance but also in efficiency. For instance, a data center powered by five DGX A100 systems can perform the work of a significantly larger traditional data center, but at a fraction of the power consumption and cost. This system is a testament to the scalability and efficiency of the A100, paving the way for more sustainable and cost-effective AI infrastructure.
AI at the Edge: Expanding A100’s Reach
The A100’s influence extends beyond traditional data centers to the realm of edge computing. NVIDIA has introduced products like the EGX A100 and EGX Jetson Xavier NX, designed to bring real-time AI and 5G signal processing to edge servers. These products exemplify how the A100 can be adapted for high-performance compute or AI workloads in smaller, more constrained environments, delivering up to 21 trillion operations per second. This adaptability is crucial as AI inferencing becomes a dominant market, especially in edge computing applications.
The A100’s Role in Diverse AI Applications
Transforming AI Services and Medical Imaging
The NVIDIA A100 GPU, with its advanced Tensor Core architecture, has significantly impacted various industries, starting from enhancing AI services to revolutionizing medical imaging. In the realm of AI services, the A100 has been instrumental in making interactions with services like Microsoft Bing more natural and efficient, delivering accurate results in less than a second. This improvement in performance is crucial for services that rely on speedy and accurate AI-driven recommendations and responses.
In the medical field, startups like Caption Health are utilizing the A100’s capabilities for crucial tasks like echocardiography, which was particularly pivotal during the early days of the COVID-19 pandemic. The A100’s ability to handle complex models, such as 3D U-Net used in the latest MLPerf benchmarks, has been a key factor in advancing healthcare AI, enabling quicker and more accurate medical imaging and diagnostics.
Automotive Industry: Advancing Autonomous Vehicles
The automotive industry, particularly in the development of autonomous vehicles (AV), has also seen substantial benefits from the A100’s AI and computing power. The iterative process of AI model development for AVs involves extensive data curation, labeling, and training, which the A100 efficiently supports. With its massive data handling and processing capabilities, the A100 is crucial for training AI models on billions of images and scenarios, enabling more sophisticated and safer autonomous driving systems.
Retail and E-Commerce: Driving Sales through AI
In the retail and e-commerce sector, AI recommendation systems powered by the A100 have made a significant impact. Companies like Alibaba have utilized these systems for events like Singles Day, leading to record-breaking sales. The A100’s ability to handle large-scale data and complex AI models is a key factor in driving these sales through personalized and effective product recommendations.
AI Inference in the Cloud
The A100’s role is not limited to training; it has also excelled in AI inference, especially in cloud environments. More than 100 exaflops of AI inference performance were delivered by NVIDIA GPUs in the public cloud in the last year, surpassing cloud CPUs for the first time. This milestone indicates a growing reliance on A100-powered GPUs across industries, including automotive, healthcare, retail, financial services, and manufacturing, for AI inference tasks.
Future-Proofing with the A100
The Evolution of AI Workloads: Preparing for the Future
The future of AI training and inference is poised to make significant strides, primarily driven by the capabilities of the NVIDIA A100. As AI models grow in complexity, addressing challenges like conversational AI, the need for powerful and efficient training and inference solutions becomes increasingly crucial. The A100, with its ability to provide up to 20X higher performance over previous generations, is designed to meet these emerging demands. Its role in accelerating diverse workloads, including scientific simulation and financial forecasting, signifies its readiness to adapt to the evolving AI landscape.
Supercomputing and AI: Pioneering the Next-Generation Infrastructure
NVIDIA’s advancements in AI supercomputing, demonstrated by the DGX A100 and DGX SuperPOD systems, are indicative of the A100’s capability to handle future AI challenges. The DGX A100, offering 5 petaflops of AI performance, and the DGX SuperPOD, with its formidable 700 petaflops, are reshaping the concept of data centers, providing scalable and efficient solutions for intensive AI workloads. These developments underscore the A100’s potential in powering the next generation of AI supercomputers, which will be essential for large-scale AI applications and complex data analyses.
AI at the Edge: The Next Frontier
The A100’s integration into edge computing applications represents a significant future trend. NVIDIA’s EGX A100 and EGX Jetson Xavier NX, designed for high-performance computing and AI workloads at the edge, illustrate the A100’s versatility. With increasing emphasis on AI inference at the edge, the A100’s ability to provide real-time AI and 5G signal processing up to 200 Gbps showcases its potential in supporting edge AI applications, which are becoming increasingly important in industries such as automotive and healthcare.
Embracing Continuous Innovation in AI
As AI continues to evolve, the A100 is well-positioned to support this growth through its integration into diverse AI infrastructure and applications. Its role in training AI models for scientific workloads and its unparalleled ecosystem, which includes partners in cloud services and system manufacturing, ensures that the A100 remains at the forefront of AI innovation. Continuous software optimizations and updates to NVIDIA’s software stack, including CUDA-X libraries and frameworks like NVIDIA Jarvis and Merlin, further enhance the A100’s capabilities, ensuring it remains a key player in future AI advancements.
The Horizon of AI: Advancements and Innovations
Advancements in AI Research and Applications
Recent developments in AI research and applications are shaping the future trajectory of the field. One significant area of progress is in the application of machine learning in natural sciences, as seen with Microsoft’s AI4Science organization. This initiative focuses on creating deep learning emulators for modeling and predicting natural phenomena, leveraging computational solutions to fundamental equations as training data. Such advancements have the potential to revolutionize our understanding of natural processes and aid in critical areas like climate change and drug discovery. For instance, AI4Science’s Project Carbonix aims to develop materials for decarbonizing the global economy, while the Generative Chemistry project collaborates with Novartis to enhance drug discovery processes.
Memory and Storage in AI Hardware
In the realm of AI hardware, memory and storage play a pivotal role in the efficient functioning of AI applications. High memory-bandwidth requirements are essential for deep neural networks, which necessitate dynamic random access memory (DRAM) to store and process data rapidly. As AI models, such as those used in image recognition, become more complex, the demand for high-bandwidth memory (HBM) and on-chip memory is increasing. These technologies allow AI applications to process large datasets quickly and with minimal power requirements. The growth in the memory market, expected to reach $12.0 billion by 2025, reflects the increasing importance of memory in AI hardware. Furthermore, AI applications are generating vast volumes of data, escalating the demand for storage solutions that can adapt to the changing needs of AI training and inference.
Potential and Ethical Considerations of AI
The potential of AI to augment and assist humans in various fields is a prominent theme in current AI research. AIs are increasingly excelling in large data regimes, especially in tasks like drug discovery, decision-making in healthcare, and autonomous driving. However, there is a growing recognition of the importance of ensuring that AI tools are ethical and free from discriminatory flaws before they become mainstream. Institutions of higher education are also focusing on preparing students to navigate the complex ethical landscape of AI, emphasizing the need for interdisciplinary dialogue and understanding of AI’s impact on society.
Innovations in AI Model Processing
NVIDIA’s continuous improvement of GPU cores, including the development of highly tuned Tensor Cores and the introduction of the Transformer Engine in Hopper Tensor Core GPUs, is a significant advancement in AI model processing. These innovations are tailored to meet the evolving needs of AI models, particularly in handling complex tasks like generative AI. The growth in AI model complexity, with current state-of-the-art models like GPT-4 featuring over a trillion parameters, necessitates these advancements in GPU technology. NVIDIA’s efforts in software development, including frameworks like NVIDIA NeMo for building and running generative AI models, also play a critical role in the evolving AI landscape.
AI’s Impact Across Various Domains
The last five years have seen major progress in AI across various sub-areas, including vision, speech recognition, natural language processing, and robotics. Breakthrough applications have emerged in domains like medical diagnosis, logistics, autonomous driving, and language translation. The use of generative adversarial networks (GANs) and advancements in neural network language models like ELMo, GPT, and BERT have been particularly notable. These developments highlight the expanding capabilities of AI and its increasingly pervasive impact on diverse aspects of life and society applications.
Newsletter
You Do Not Want to Miss Out!
Step into the Future of Model Deployment. Join Us and Stay Ahead of the Curve!