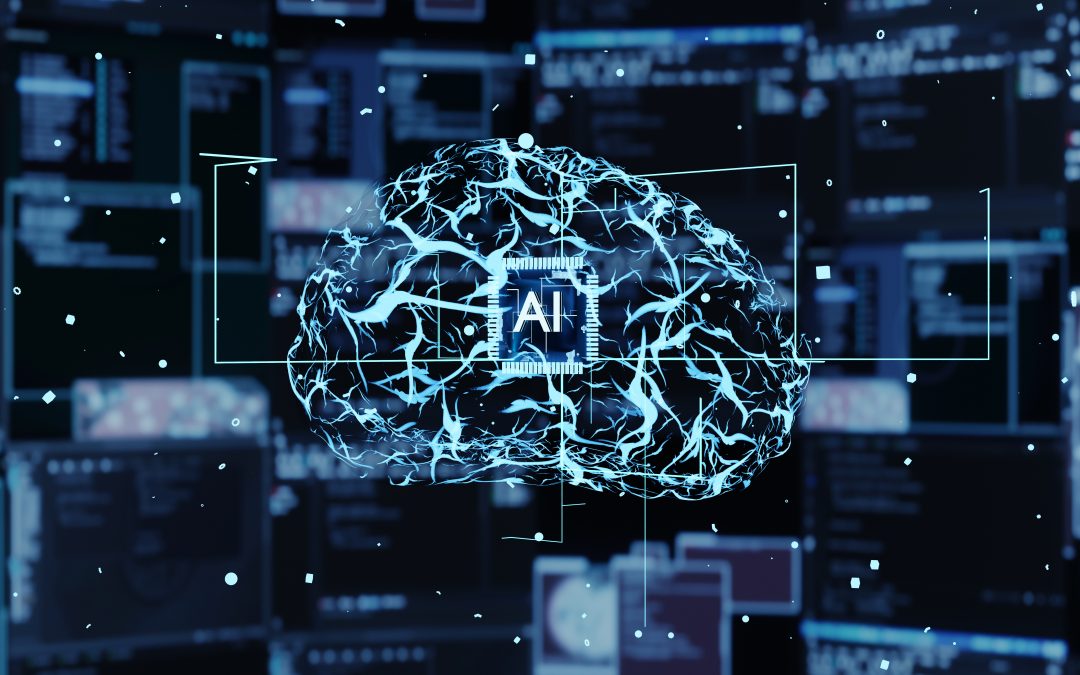
Inference in Scientific Research
Inference in Scientific Research
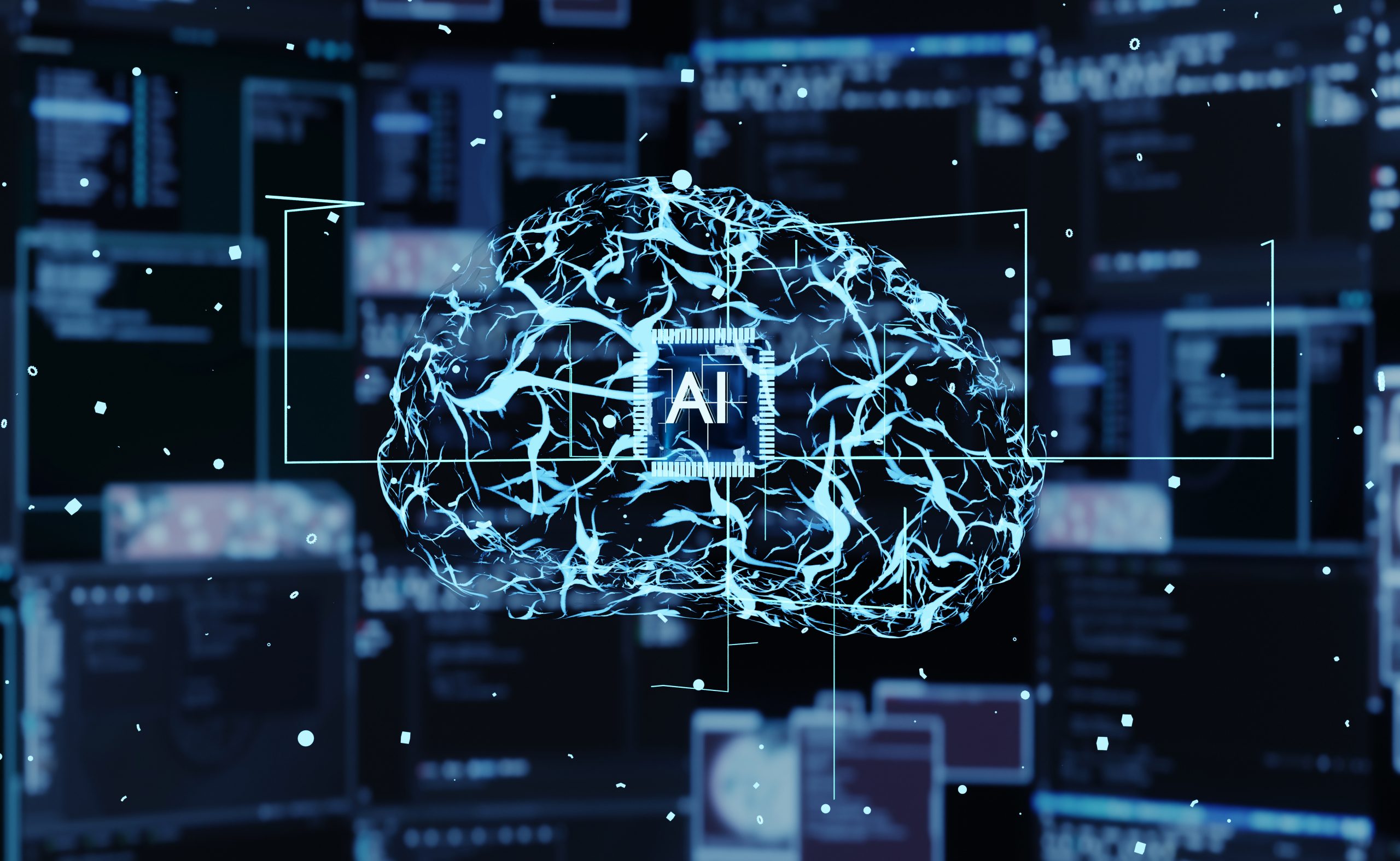
Introduction to Inference in Scientific Research
Scientific inquiry is an intricate tapestry woven with various threads of reasoning, experimentation, and interpretation. At its core lies the fundamental process of scientific inference, a methodological cornerstone that turns data into discoveries. This process is the alchemy that transforms raw observations into refined knowledge, guiding scientists through the labyrinth of the unknown to arrive at logical, evidence-based conclusions.
Inference in scientific research is akin to the art of reading between the lines of nature’s complex narrative. It is the intellectual process by which scientists, equipped with fragments of information and observational shards, construct a coherent picture of the underlying phenomena. This journey from observed data to conceptual understanding involves a delicate balance between empirical evidence and theoretical frameworks. It’s a dance between what is seen and what is unseen, where the unseen is illuminated by the seen.
The essence of scientific inference is not just about making guesses; it is about making informed, logical deductions that are rooted in empirical evidence. This process is driven by a blend of creativity and rigor, intuition and analysis, hypothesis and experiment. It is a dynamic process, constantly evolving with the acquisition of new data, the refinement of techniques, and the advancement of theoretical understanding.
In this section, we will embark on an exploration of the nuanced facets of scientific inference. We will delve into its essential role in the scientific method, unravel its intricate relationship with data and theory, and illuminate how it bridges the gap between observation and understanding. Our journey will reveal the subtle artistry and profound logic that underpin this vital component of scientific exploration, offering insights into the very fabric of scientific discovery.
Understanding Inference: Inductive vs Deductive
In the realm of scientific inference, two primary methodologies – inductive and deductive reasoning – serve as the backbone of research and discovery. These methods, while distinct, are not mutually exclusive and often work in tandem to advance our understanding of the world.
Inductive Inference: From Observation to Theory
Inductive reasoning is the Sherlock Holmes of scientific methods. It begins with observations, often specific and detailed, leading to broader generalizations and theories. This method is akin to putting together a jigsaw puzzle; each piece of data is an individual part of a larger picture. The process involves three key stages: observation, pattern identification, and theory development. For instance, observing a specific trend in climate change data over decades might lead to a generalized theory about global warming.
However, inductive conclusions, no matter how logical they may seem, are not definitive proofs but rather informed suppositions. They are always open to challenge and revision with new evidence, highlighting the provisional nature of scientific knowledge.
Deductive Inference: From Theory to Confirmation
Conversely, deductive reasoning is the process of starting with a general theory or hypothesis and working towards specific, testable conclusions. It is a top-down approach where the focus is on testing and validating existing theories rather than generating new ones. The deductive method is methodical and structured, involving hypothesis formulation, data collection, and analysis to confirm or refute the hypothesis. This approach is exemplified in controlled experiments in laboratories, where variables are manipulated to test a theory’s predictions.
The strength of deductive reasoning lies in its ability to provide conclusive results, but its reliability is contingent on the accuracy of the initial assumptions or theories. If the foundational theory is flawed, the deductive conclusions drawn from it will be equally suspect.
In summary, inductive reasoning is characterized by its exploratory nature, generating new theories from specific observations. Deductive reasoning, in contrast, rigorously tests these theories to confirm their validity. Both are essential to the scientific method, providing a dynamic interplay between theory and observation, speculation and evidence.
The Process and Importance of Inference in Scientific Studies
In the expansive landscape of scientific research, inference serves as a compass, guiding researchers through the complex terrain of data interpretation and hypothesis testing. This section explores the integral role of inference in shaping experimental design and facilitating scientific discoveries.
Crafting the Blueprint: The Role of Inference in Experimental Design
At the heart of scientific exploration lies the experimental design, a meticulously crafted blueprint for discovery. Here, inference is pivotal, acting as the architect that shapes the structure of research. Experimental design is fundamentally about testing hypotheses – conjectures informed by inference from previous observations or established theories. This process requires a deep understanding of the subject at hand, transforming abstract concepts into testable predictions.
Designing an experiment is a multi-faceted process, encompassing variable consideration, hypothesis formulation, treatment design, subject assignment, and measurement planning. Inference plays a critical role in each of these steps, guiding researchers in establishing relationships between variables, formulating hypotheses, and planning measurements. It is the thread that weaves together various elements of an experiment, ensuring coherence and relevance to the research question.
Balancing the Scales: Inference and the Pursuit of Validity
One of the greatest challenges in scientific research is achieving valid and reliable conclusions. Inference is key in balancing this scale. Selecting a representative sample and controlling extraneous variables are fundamental to this process. Random assignment of participants to control and treatment groups, a cornerstone of experimental integrity, is often guided by inferential statistics. When random assignment is impractical, researchers may turn to observational studies, where inference aids in minimizing biases such as sampling, survivorship, and attrition.
Unseen Threads: The Invisible Influence of Inference
Inference, though not always overtly recognized, is an unseen force permeating every aspect of scientific research. It informs decisions at each stage of the scientific process, from preliminary observation to final analysis. The strength of scientific conclusions is often a reflection of the quality of inferences drawn throughout the research process.
In summary, inference in scientific studies is not just a step in the process; it is the backbone that supports the entire scientific endeavor. From designing experiments to drawing conclusions, inference is the guiding light that leads researchers through the intricate maze of scientific inquiry, ensuring that each step is grounded in logic, evidence, and a deep understanding of the phenomena under study.
Case Studies: Inference in Action
The art of inference in scientific research is best illustrated through tangible examples where its application has led to significant breakthroughs or shaped our understanding of complex phenomena. These case studies reveal the profound impact of inference on scientific progress.
Hypothesis Formulation in Research: The Flu Vaccine Study
One exemplary case of inference in scientific research is seen in the study of flu vaccines. Researchers aimed to determine the vaccine’s effectiveness in reducing flu cases in the general population. Due to the impracticality of studying the entire population, a representative sample was used to make inferences about the vaccine’s impact. The study followed a specific methodology: selecting a representative sample, measuring relevant variables, and using statistical methods to generalize the sample results to the population. The findings, initially based on sample data, were substantiated through hypothesis testing and confidence intervals, providing credible evidence for the vaccine’s effectiveness in the broader population.
Real-world Applications of Inference: Newton’s Universal Gravitation
Isaac Newton’s argument for universal gravitation exemplifies the role of inference in developing foundational scientific theories. Newton’s methodological approach, as outlined in his “Principia,” combined empirical observations with a set of methodological rules emphasizing simplicity and inductive generalization. By inferring common causes for observed phenomena, such as the behavior of planetary bodies and their satellites, Newton formulated the principle of universal gravitation. This principle posited gravity as a mutually attractive force acting on all bodies, fundamentally changing our understanding of physics and the universe. Newton’s inferences, grounded in simplicity and empirical evidence, illustrate how scientific inference can lead to profound and enduring theories.
The Interplay of Observation and Theory: Copernican Heliocentrism
Another pivotal moment in scientific history where inference played a crucial role was during the Copernican Revolution. Nicolaus Copernicus, through his heliocentric model of the solar system, challenged the prevailing geocentric model. Copernicus’ model was not initially more accurate in predicting celestial motions than the geocentric model. However, its relative simplicity and coherence with observed phenomena gradually led to its acceptance. This shift was a result of inferential reasoning, where the simplicity and explanatory power of the heliocentric model were inferred to be more plausible and closer to the truth than the complex and cumbersome Ptolemaic system.
In conclusion, these case studies demonstrate the indispensable role of inference in scientific research. From formulating hypotheses in contemporary studies to shaping foundational scientific theories, inference serves as a key tool in the scientist’s arsenal, enabling the leap from observation to understanding, from data to discovery.
Challenges and Limitations in Scientific Inference
The process of inference in scientific research, while powerful, is not without its challenges and limitations. Understanding these constraints is crucial for maintaining the integrity and reliability of scientific findings.
Testability and Falsifiability: Essential Yet Limiting
A fundamental limitation of scientific inference is rooted in the very nature of the scientific method: the requirement for hypotheses to be testable and falsifiable. This criterion, while essential for scientific rigor, inherently places certain topics beyond the reach of scientific inquiry. Phenomena or hypotheses that cannot be empirically tested or potentially disproven are not amenable to scientific investigation. This limitation delineates the boundary of scientific exploration, ensuring focus on verifiable and refutable propositions, but also excluding certain areas of inquiry that cannot be addressed through empirical means.
The Inability to Address Non-Empirical Realms
Scientific inference is incapable of proving or refuting the existence of entities or phenomena that fall outside empirical observation. For instance, the existence of supernatural entities or divine powers remains outside the purview of scientific inquiry. Attempts to apply scientific principles to such concepts, as seen in debates around ideas like intelligent design, highlight the limitations of scientific inference in addressing questions that are fundamentally non-empirical. This underscores the importance of distinguishing between empirical scientific theories and philosophical or theological assertions.
Science and Value Judgments
Another key limitation is science’s inability to make value judgments. Scientific inference can study the causes and effects of phenomena like global warming but cannot assert normative statements about them. The use of scientific data to advance moral or ethical positions often leads to the blurring of lines between objective science and subjective values. This can result in the creation of pseudo-science, where scientific claims are used to legitimize untested or untestable ideas, distorting the essence of scientific inquiry.
The Coexistence of Competing Theories
In certain instances, scientific inference leads to situations where competing theories coexist to explain a single phenomenon. A classic example is the dual nature of light, which exhibits properties of both waves and particles. This duality challenges the simplistic view of scientific inference that leads to a single explanatory theory. Instead, it showcases the complexity of natural phenomena and the nuanced nature of scientific understanding.
In summary, while scientific inference is a powerful tool for understanding the natural world, it operates within certain constraints. These limitations, inherent in the nature of scientific inquiry, shape the scope and reliability of the conclusions drawn from scientific research.
The Role of Technology and Data Analysis in Enhancing Inference
The interplay between scientific inference and technology is a symbiotic one, where each propels the other forward, leading to advancements in both domains. The impact of technology and data analysis in enhancing scientific inference is multifaceted and profound.
Technology as a Catalyst for New Scientific Observations
The advancement of technology has historically enabled new scientific discoveries. For instance, the invention of the cathode ray tube in the 1800s led to the discovery of electrons, the atomic nucleus, and X-rays. These discoveries, in turn, catalyzed further technological innovations, such as the development of the X-ray machine and CT scan machines, revolutionizing medical diagnostics and opening new frontiers in fields like archaeology and paleontology.
X-ray Crystallography: Bridging Technology and Molecular Science
A noteworthy example of technology augmenting scientific inference is X-ray crystallography. This technique, stemming from the discovery of X-rays, allows scientists to deduce the arrangement of atoms in a crystal by analyzing how X-rays are diffracted through it. This method has profoundly influenced science by providing detailed images of molecular structures, leading to significant advancements in fields ranging from biology to materials science.
DNA Research: The Interplay of Scientific Discovery and Technological Progress
The discovery of DNA’s structure is another instance where technological advancements and scientific inference have intertwined. Understanding DNA’s structure has led to the development of polymerase chain reaction (PCR) technology, enabling the amplification of small DNA samples. This technology has had wide-ranging implications, from advancing criminal forensics through DNA fingerprinting to propelling research in genetics and biotechnology.
In conclusion, technology and data analysis play a crucial role in enhancing scientific inference. They not only provide the tools and methodologies necessary for exploring new frontiers of knowledge but also contribute to refining and expanding the scope of scientific inquiry itself.