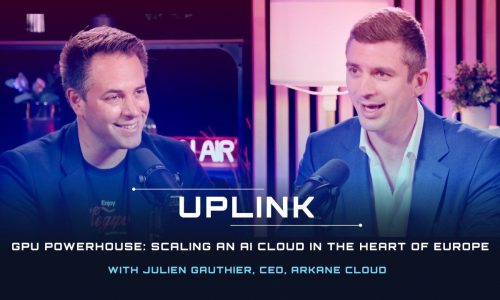
GPU Rental for Machine Learning and AI Development
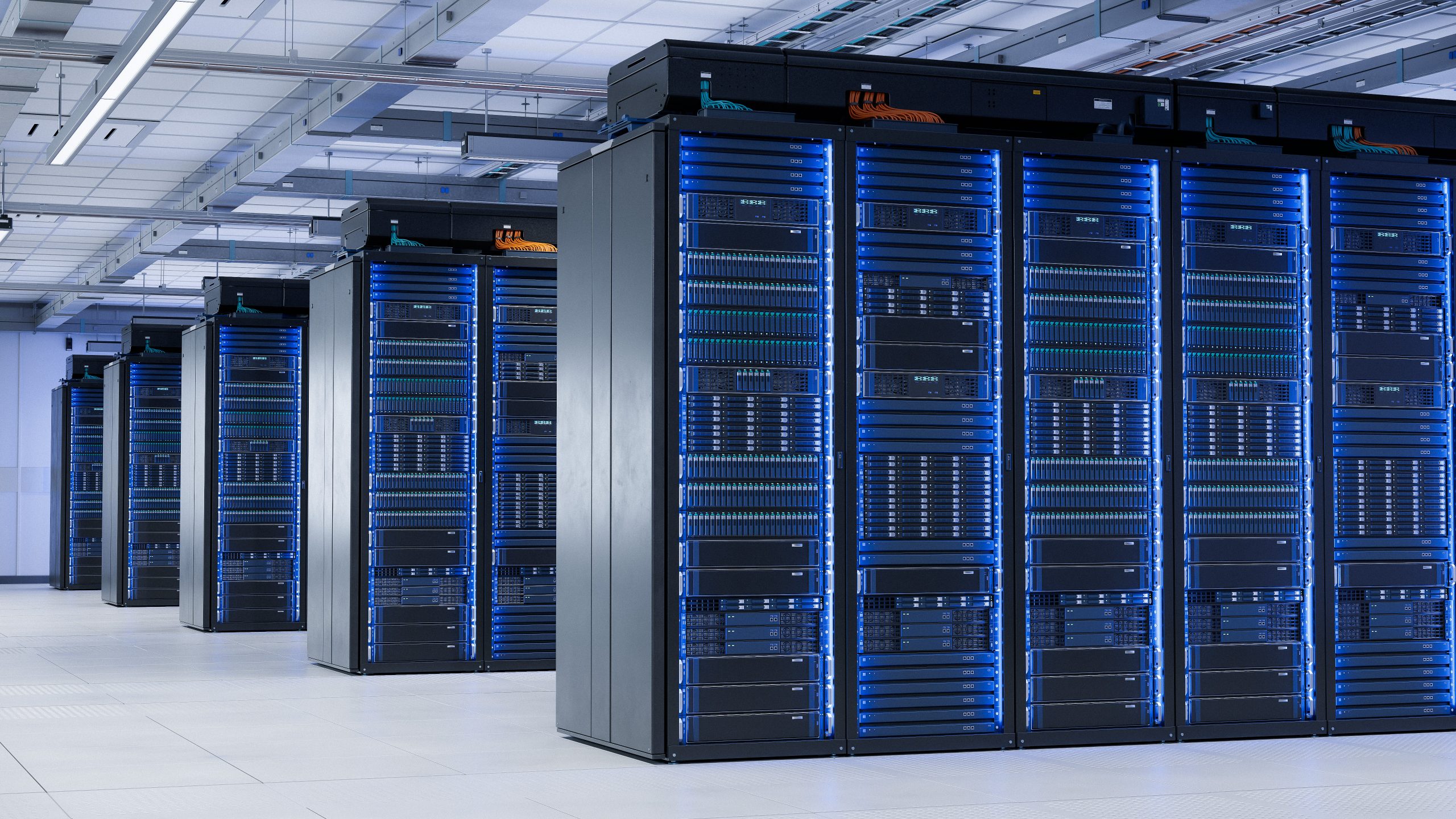
Introduction
The landscape of machine learning (ML) and artificial intelligence (AI) is rapidly evolving, driven by significant advancements in technology and increasing computational demands. At the forefront of this evolution are Graphics Processing Units (GPUs), which have become essential for the efficient processing of complex algorithms and large datasets intrinsic to ML and AI. This section explores the current state of AI, highlighting the transformative role GPUs play in this field.
Artificial intelligence technologies are reshaping business processes and societal structures, with AI trends in 2023 reflecting a focus on the achievements and advancements of algorithms. Tools that automate ML pipelines are significantly accelerating development processes, making GPUs indispensable for enterprises engaging in AI and ML projects. Innovations in AI are expanding into various domains such as conceptual design, smaller devices, and multi-modal applications. This diversification necessitates a more robust and adaptable computational infrastructure, where GPUs play a critical role.
One of the key trends in AI and ML is Automated Machine Learning (AutoML). It involves improved tools for labeling data and automatically tuning neural network architectures. AutoML streamlines the process of developing AI models, making it more efficient and cost-effective. This trend underscores the growing need for high-performance GPUs capable of handling the intensive computational requirements of these tasks.
Multi-modal learning, another significant trend, involves combining multiple modalities within a single ML model, such as text, vision, speech, and IoT sensor data. This approach requires powerful computational resources to process and analyze diverse data types effectively. GPUs provide the necessary horsepower to support these sophisticated models, enabling advancements in fields like healthcare, where AI algorithms can optimize the presentation of complex patient data, thereby improving medical diagnosis.
Moreover, the demand for improved language modeling, as demonstrated by AI models like ChatGPT, is on the rise. These models require robust GPUs to process vast amounts of data and deliver accurate, interactive AI experiences across various fields. The push for quality control in AI language models further amplifies the need for reliable, high-performance GPUs.
Lastly, democratized AI, which lowers the expertise barrier for building AI models, is gaining traction. This trend emphasizes the need for accessible, high-performance computational resources like GPUs. By democratizing AI, more individuals and organizations can participate in AI development, fostering innovation and broadening the scope of AI applications.
In summary, the current trends in AI and ML underscore the critical role of GPUs in advancing these technologies. As AI continues to permeate various industries and applications, the demand for powerful, flexible, and cost-effective GPU solutions will only increase, making GPU rental an attractive option for developers and companies engaged in AI and ML projects.
The Rising Demand for GPUs in AI and ML
The integration of Graphics Processing Units (GPUs) in artificial intelligence (AI) and machine learning (ML) has been transformative, setting a new benchmark for computational capabilities. GPUs, initially designed for rendering graphics, have evolved into vital tools for AI and ML development, offering unparalleled efficiency in processing complex algorithms and large datasets.
The Foundational Role of GPUs in AI
GPUs have become the bedrock of AI technologies, particularly in deep learning. Their ability to process large neural networks has brought solutions like autonomous driving and facial recognition into the mainstream. The pivotal role of GPUs in high-performance computing, a key component of cloud computing, enables tasks previously reserved for supercomputers. This shift has made advanced computing more accessible and cost-effective.
Understanding GPU Functionality
The architecture of GPUs, optimized for parallel processing, allows them to handle math-heavy computations effectively. This capability is crucial for applications requiring the processing of high-resolution images or videos, where GPUs vastly outperform CPUs. For instance, a task that would take several years on a single CPU can be completed within a day using a few GPUs. This remarkable performance is a game-changer for projects requiring heavy computational power.
Evolution and Integration of GPUs
GPUs have expanded their utility beyond graphics processing to encompass AI and other data-intensive tasks. The introduction of CUDA by NVIDIA in 2006 was a significant milestone, enabling developers to efficiently use GPUs for parallel computing. This advancement facilitated the partitioning of complex problems into smaller, manageable tasks, enhancing the efficiency of AI model development.
Furthermore, NVIDIA’s partnership with Red Hat OpenShift to integrate CUDA with Kubernetes has streamlined the development and deployment of applications on GPU servers. This collaboration simplifies the process of utilizing GPUs in data science workflows, exemplified by the Red Hat OpenShift Data Science (RHODS) platform. RHODS allows users to customize GPU resources for their data mining and model processing tasks, further democratizing access to high-powered computing.
In conclusion, the rising demand for GPUs in AI and ML is driven by their unparalleled computational power, flexibility, and efficiency in handling complex, data-intensive tasks. As AI and ML continue to evolve, GPUs will remain central to these advancements, offering a robust foundation for innovation and development in various fields.
Cost Efficiency of GPU Rental
In the rapidly advancing fields of artificial intelligence (AI) and machine learning (ML), the economic implications of GPU usage are a significant consideration. Renting GPUs offers a cost-effective and flexible alternative to purchasing, especially relevant for AI development.
High Cost of GPU Ownership
Purchasing GPUs for AI and ML tasks represents a substantial financial investment. GPUs, essential for compute-intensive tasks like deep learning, have a wide price range, with some models like h100 costing upwards of $30,000. This high cost, combined with the rapid advancement in GPU technology leading to quick obsolescence, makes purchasing GPUs a considerable long-term financial commitment. The value of GPUs does not appreciate over time, with a typical GPU losing about 15% of its value each year. Therefore, owning GPUs can be economically burdensome due to initial high costs, depreciation, and associated maintenance expenses.
Flexibility and Scalability in Renting
GPU rental provides flexibility, allowing users to rent high-performance hardware for short periods. This flexibility is ideal for projects with varying computational needs or those in experimental stages. Users can rent GPUs matching their specific requirements and adjust the scale of resources as their project evolves, without the long-term commitment and financial burden of a purchase. Renting GPUs is particularly beneficial for small-scale projects, where the high cost of purchasing may not be justifiable. Moreover, rented GPUs can be updated or changed easily as newer models become available, ensuring access to the latest technology without additional investment.
Cost-Effective Solution for Diverse Users
Renting GPUs is more economical than buying, as it avoids the upfront expense of purchasing hardware. This cost advantage is particularly significant for AI developers, researchers, startups, and small businesses, who can access powerful computing resources at a fraction of the cost. For instance, services like Arkane Cloud offer GPU rentals at competitive rates, significantly lowering the financial barrier for accessing high-performance computing. This affordability enables a broader range of users, including those with limited budgets, to leverage GPU computing for AI development, testing, and optimization without compromising performance.
Practical Considerations in Renting vs. Purchasing
The decision to rent or purchase GPUs also hinges on specific project requirements, such as the type of models being trained, electricity costs, and personal research preferences. For example, Arkane Cloud services illustrate that once an AI model is trained using rented GPUs, the model can be exported and run on less expensive hardware, eliminating the need for a high-powered machine post-research. Additionally, the total cost of ownership, including the purchase price and ancillary costs like electricity and maintenance, often makes renting a more viable option financially. Renting GPU servers, therefore, emerges as a practical and cost-effective solution for a wide range of AI and ML projects.
Flexibility and Scalability
The flexibility and scalability of GPU rental for AI and machine learning projects are increasingly recognized as critical advantages, particularly in a field characterized by rapid technological advancements and varying computational needs.
Adaptive Resource Allocation
Renting GPUs provides the flexibility to adapt resource allocation to the specific requirements of a project. This adaptability is crucial in AI and ML where computational needs can fluctuate. Renting allows for the selection of GPUs that are best suited for specific tasks and the ability to scale resources up or down as project demands change. This flexibility is particularly beneficial for projects at different stages of development or for those that require short-term, intensive computational power.
Access to High-Performance Hardware
Another significant advantage of GPU rental is the opportunity to access high-performance hardware that might be beyond the budget constraints if purchased outright. Renting enables the use of powerful GPUs with unique characteristics and configurations that are essential for complex AI and ML tasks but might be prohibitively expensive to buy. This access not only aids in maintaining a competitive edge in AI and ML projects but also ensures that the latest technology is always within reach without the need for continual hardware updates or replacements.
Cost-Effective Experimentation
GPU rental is ideal for experimentation and testing in AI and ML. It allows researchers and developers to try different GPUs for specific tasks without committing to a purchase. This ability to “try before you buy” is particularly advantageous in a field where the right hardware can significantly impact the success of a project. Renting GPUs as needed for short-term projects or experiments avoids the long-term financial commitment and potential underutilization associated with purchasing GPUs.
Keeping Up with Technological Advancements
In the fast-paced world of AI and ML, staying abreast of technological advancements is crucial. GPU rental services provide the agility to keep up with these changes. They offer a way to access the latest GPU technology as it becomes available, without the need for significant reinvestment in new hardware. This approach not only saves time and resources but also ensures that AI and ML projects are always powered by the most current and capable technology available.
In summary, the flexibility and scalability offered by GPU rental are indispensable in the dynamic landscape of AI and ML. They provide a cost-effective, adaptable, and technologically current solution to meet the varying and evolving computational needs of these fields.
Staying Technologically Current
In the dynamic realm of AI and machine learning, staying technologically current is crucial. GPU rental offers a unique advantage in this regard, allowing users to access the latest hardware without the financial burden and inflexibility associated with purchasing.
Access to the Latest Technology
One of the foremost benefits of renting GPUs is the ability to use more powerful and advanced GPUs than what might be financially feasible to purchase. This access is particularly beneficial in a field that rapidly evolves, where staying updated with the latest advancements is vital for maintaining a competitive edge. Renting also allows access to GPUs with unique characteristics, such as specialized processor configurations or multiple cards in a single system, which might not be available or affordable in the consumer market. This flexibility ensures that users can always utilize the most suitable and advanced technology for their specific AI and ML projects.
Future-Proofing Projects
The fast pace of technological advancements in GPU technology means that newer, more capable models are continually being released. Renting GPUs circumvents the issue of hardware becoming outdated quickly. It offers a way to keep up with the latest trends and technology without the need to resell old hardware to finance new purchases. This approach effectively future-proofs projects, ensuring that they benefit from the latest advancements without the risks and costs associated with owning rapidly aging technology.
Reducing Research and Decision Time
Choosing the right GPU for specific AI and ML tasks can be daunting, especially given the fast-evolving nature of technology and the plethora of options available. Renting GPUs simplifies this process. Rental companies typically do extensive research to offer the best and most suitable options to their customers. This service saves users significant time and effort that would otherwise be spent researching various GPU models, reading reviews, and comparing specifications. With rental services, making an informed decision about which GPU to use becomes a matter of a few clicks, significantly streamlining the process of acquiring the right technology for specific needs.
In conclusion, GPU rental offers an adaptable, cost-effective, and efficient way to stay technologically current in the fast-paced world of AI and ML. It provides access to the latest hardware, ensures projects are future-proofed against rapid technological advancements, and simplifies the decision-making process, all of which are crucial for driving innovation and maintaining competitiveness in these fields.
Enhanced Performance and Efficiency
The impact of GPU rental on performance and efficiency in AI and machine learning is profound, addressing the growing demand for high-performance computing in these fields.
Accelerating Deep Learning Processes
Deep learning, a critical subset of machine learning, relies heavily on GPUs for their ability to perform parallel computations. This capability is crucial for training complex neural networks, a staple in AI development. Cloud GPUs, in particular, have become indispensable for deep learning, offering performance speeds up to 250 times faster than traditional CPUs. This dramatic increase in speed significantly accelerates the training of AI models, rendering intricate 3D graphics, and even gaming applications, making GPUs a cornerstone in modern technology.
Scalability Advantages
One of the key benefits of cloud GPU rental is scalability. As AI and ML projects evolve, computational needs can fluctuate. Cloud GPUs offer the flexibility to scale resources up or down according to these changing demands. This scalability is vital for growing organizations, ensuring that their computational infrastructure can adapt to their evolving needs without the constraints posed by physical GPUs.
Cost-Effectiveness
Renting GPU on the cloud provides a more cost-effective solution compared to the substantial investment required for purchasing high-powered GPUs. The pay-as-you-go model significantly reduces costs, particularly for organizations and projects that do not require constant access to advanced GPU resources. This economic efficiency is especially crucial for startups and smaller businesses venturing into AI and ML, as it enables them to leverage high-performance computing without hefty initial investments.
Space and Time Efficiency
In addition to performance benefits, cloud GPUs offer space and resource efficiency. Unlike physical GPUs, which occupy significant space and resources on local machines, cloud GPUs operate remotely. This setup allows local computers to be utilized for other tasks, offloading heavy computational work to the cloud. Moreover, the power of cloud GPUs can drastically reduce the time required to complete complex tasks, translating into higher productivity and more opportunities for innovation.
In summary, GPU rental enhances both performance and efficiency in AI and ML projects. It accelerates deep learning processes, offers scalable resources, provides cost-effective solutions, and improves space and time efficiency. These benefits are crucial for driving forward the advancements in AI and ML, making GPU rental an attractive option for a wide range of users and organizations.
Real-World Applications and Use Cases
The real-world applications of GPU rental in AI and ML are diverse, spanning various industries and revolutionizing conventional processes.
Transformative Applications Across Industries
AI, powered by advanced ML tools like Deep Reinforcement Learning, Generative Adversarial Networks, and Natural Language Processing, is reshaping global productivity and lifestyles. The success of these AI and ML systems largely depends on their ability to process vast amounts of data using GPUs. These applications have led to substantial improvements in industries like manufacturing, autonomous driving, and healthcare, where GPU-powered AI is driving advancements and operational efficiency.
Autonomous Driving
In autonomous driving, GPUs play a pivotal role in processing the extensive sensor data collected by vehicles. This data includes visual information from cameras, LiDAR readings, and other sensor inputs. The computational power of GPUs is crucial for real-time object detection, classification, and motion detection, enabling autonomous vehicles to make split-second decisions critical for safety and navigation.
Healthcare and Medical Imaging
In healthcare, especially in medical imaging, GPUs assist in processing complex visual data, aiding in the detection of rare diseases and conditions. Their ability to handle large, data-intensive tasks is instrumental in supporting the decision-making process of radiologists and clinicians, particularly in cancer detection and other critical diagnoses where traditional computational methods fall short.
Fighting Disease and Medicine Discovery
GPU rental has also shown its potential in fighting diseases and aiding medicine discovery. For instance, in the development of vaccines for viruses like COVID-19, GPU-powered AI systems like AlphaFold have made significant contributions by predicting the 3-D structure of proteins based on genetic data. This advancement has been instrumental in understanding viruses and developing effective treatments.
Environmental and Climate Science
In environmental and climate science, the challenges posed by climate change require robust predictive models and high-fidelity data visualization, areas where GPUs excel. They support complex weather prediction and climate modeling efforts, enabling scientists to tackle Earth-scale problems that are otherwise intractable with current hardware and software capabilities.
Smart Manufacturing
In the manufacturing sector, the introduction of AI and ML, supported by GPUs, has led to the era of smart manufacturing. Deep learning technologies are being utilized for various purposes, including design, quality control, machine/process optimization, supply chain management, and predictive maintenance. GPUs facilitate the processing of the massive data volumes generated in modern manufacturing environments, helping businesses make sense of complex information and improve operational efficiency.
In conclusion, the versatility of GPU rental in AI and ML projects is evident across multiple sectors. Its ability to handle data-intensive tasks efficiently and cost-effectively makes it a valuable asset in driving innovation and enhancing performance in diverse applications.
Newsletter
You Do Not Want to Miss Out!
Step into the Future of Model Deployment. Join Us and Stay Ahead of the Curve!