![The 4 Major Corporate Fears About AI [and How Arkane Crushes Them]](https://arkanecloud.com/wp-content/uploads/elementor/thumbs/image-fears-r83x02fmwkg0873grkx2f5jvdsy2k1b00h5jqz1vi0.png)
How to setup Stable diffusion
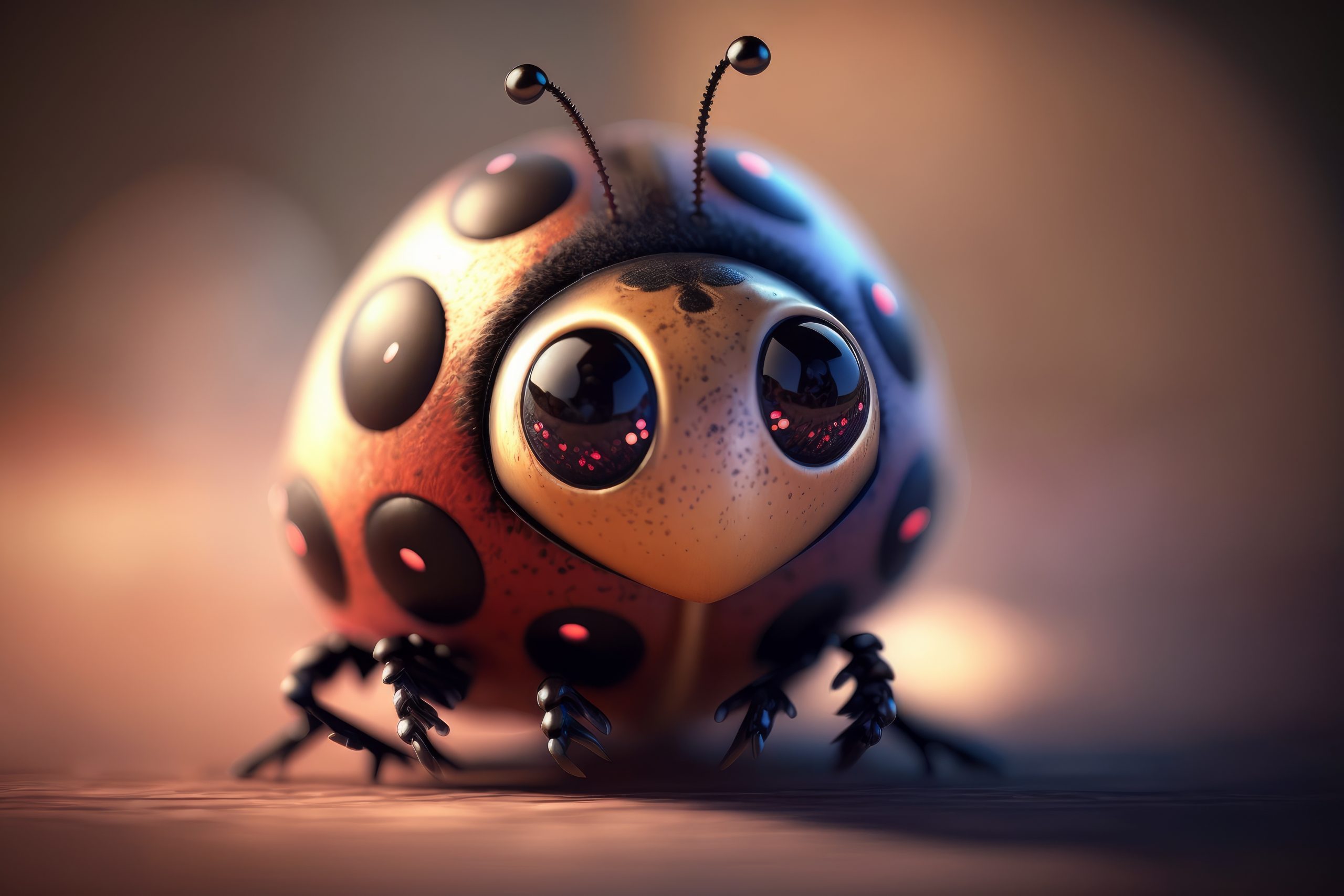
Introduction to Stable Diffusion
The landscape of artificial intelligence (AI) is continually evolving, with significant strides being made in generative AI models. A notable addition to this realm is Stable Diffusion, an open-source model renowned for its capability to generate vivid, high-quality images from textual descriptions. This technology, developed by Stability AI, has quickly become a pivotal tool in various domains, from graphic design to data augmentation.
Stable Diffusion stands out for its versatility and accessibility. It can generate images from text, modify existing images based on textual input, and enhance low-resolution images. The foundation of Stable Diffusion lies in its ability to understand and interpret textual descriptions, transforming them into visually compelling content. This is not just a mere translation of text to image but an intricate process where the AI understands context, style, and subtleties of the input to create something visually coherent and often stunning.
The most recent advancement in this technology is the introduction of Stable Video Diffusion. This new model extends the capabilities of the original image model to the realm of video, allowing for the generation of short video clips based on image-to-video models. Stable Video Diffusion, currently in its research preview phase, is particularly notable for its adaptability to various video applications. It can perform tasks like multi-view synthesis from a single image, and is being developed to support a wide range of models building on this base technology.
The performance of Stable Video Diffusion is impressive, capable of generating 14 to 25 frames at customizable frame rates, which showcases its potential in various sectors including advertising, education, and entertainment. While still in the development stage and not intended for real-world or commercial applications yet, this model exemplifies the ongoing advancements in AI and its potential to revolutionize how we interact with and conceive digital content.
Stable Diffusion, both in its original and video form, is a testament to the ongoing pursuit of enhancing and expanding the capabilities of AI models. It represents a significant step forward in the journey toward creating more versatile and accessible tools for various applications, thus amplifying human creativity and innovation.
Prerequisites for Running Stable Diffusion
Navigating the technical requirements to run Stable Diffusion is a crucial first step in leveraging this advanced AI tool for image generation. As an evolving platform, Stable Diffusion’s system needs have diversified, accommodating a wider range of hardware capabilities. Initially, running Stable Diffusion effectively required robust hardware, including 16GB of RAM and an Nvidia graphics card with at least 10GB of VRAM. This setup was well-suited for creating high-resolution images and engaging in intensive generative tasks.
However, the landscape has shifted with the advent of various forks and iterations of the tool. These have broadened the hardware spectrum, reducing the barrier to entry. Presently, the general system requirements include a Windows, MacOS, or Linux operating system, paired with a graphics card boasting a minimum of 4GB of VRAM. Additionally, at least 12GB of installation space is recommended, ideally on an SSD. It’s essential to note that these are baseline requirements. Generating images larger than 512 x 512 pixels or of higher quality demands more potent hardware.
For those inclined towards AMD graphics cards, while not officially supported, there exist specific forks that cater to these GPUs. These versions require a more intricate installation process but can harness the power of recent AMD graphics cards, especially those with 8GB of VRAM or more, to run Stable Diffusion effectively.
Intel graphics cards follow a similar narrative. They are not officially supported, yet certain forks, like OpenVino, enable users to utilize Intel Arc graphics cards for running Stable Diffusion, with superior performance noted on higher-end models.
In the realm of Apple technology, the M1 processors have their dedicated fork, InvokeAI, offering full support. This fork stipulates a minimum of 12GB of system memory and equivalent installation space, promising higher resolution and more precise image generation with more powerful M1 chip variants.
Lastly, for those without a dedicated GPU, running Stable Diffusion is still feasible. Options like DreamStudio provide an online platform for using Stable Diffusion with no hardware prerequisites. Alternatively, CPU-only forks like OpenVino offer a pathway to use the tool without a GPU, albeit with a trade-off in processing speed and efficiency.
Running Stable Diffusion Online
In the dynamic world of generative AI, the capability to run models like Stable Diffusion online is revolutionizing how professionals and enthusiasts interact with AI technology. The latest developments in online platforms for Stable Diffusion have significantly enhanced user experience and expanded creative possibilities.
The release of Stable Diffusion v2.1 marked a significant step forward. This version, powered by a new text encoder (OpenCLIP), developed by LAION, offers a more profound range of expression than its predecessor. It supports both new and old prompting styles, indicating a more versatile approach to user interactions. The dataset used for training this version is more diverse and wide-ranging, boosting image quality across various themes like architecture, interior design, wildlife, and landscape scenes. This diversification in datasets has been balanced with fine-tuned filtering for adult content, making it a robust model for a wider audience.
Stable Diffusion v2.1 also brings enhancements in image generation, especially in rendering non-standard resolutions. This capability allows users to work with extreme aspect ratios, facilitating the creation of broad vistas and widescreen imagery. Such features are particularly beneficial for professionals in fields like advertising and digital art, where visual impact and uniqueness are paramount.
Another innovative feature in v2.1 is the introduction of “negative prompts.” These prompts enable users to specify what they do not want to generate, effectively eliminating unwanted details in images. For instance, appending “| disfigured, ugly:-1.0, too many fingers:-1.0” to a prompt can correct common issues like excess fingers or distorted features. This advancement empowers users with greater control over image synthesis, allowing for more refined and precise outputs.
Looking ahead, Stability AI, the team behind Stable Diffusion, is committed to developing and releasing more models and capabilities as generative AI continues to advance. This open approach to AI development promises exciting new possibilities and enhancements for online platforms, ensuring that Stable Diffusion remains at the forefront of accessible and powerful AI tools.
Setting Up Stable Diffusion Locally
Setting up Stable Diffusion locally opens the door to a realm of creative and practical applications, far beyond basic image generation. By harnessing the power of this AI model within a local environment, developers and tech enthusiasts can embark on innovative projects, tailor-made to their specific needs and interests.
One intriguing application is the creation of AI Avatars. Utilizing Stable Diffusion, users can generate realistic avatars for various purposes, from social media profiles to character models in video games. This application not only enhances personalization but also feeds into the growing demand for unique digital identities in virtual spaces.
Another area ripe for exploration is the generation of NFT (Non-Fungible Token) collections. Artists and photographers can leverage Stable Diffusion to create distinctive AI-generated images, transforming them into digital assets for showcasing and selling online. This approach opens new avenues for digital art commerce, blending creativity with blockchain technology.
In communication, a chat extension powered by Stable Diffusion can revolutionize how we express thoughts and ideas. Imagine an app that generates images based on textual input, enabling users to convey their ideas visually during a chat. This could add a new dimension to digital communication, making it more expressive and engaging.
For content creators, an automated blog cover generator could be a game-changer. By creating images that reflect the title and content of a blog post, Stable Diffusion can help bloggers make their content stand out with visually appealing and relevant cover images.
Video content creation is another domain where Stable Diffusion’s local setup could shine. An app that generates YouTube videos based on text prompts can assist in creating educational or entertainment content, streamlining the video production process.
Furthermore, a gif generator app utilizing Stable Diffusion can add fun and creativity to digital interactions. Users could create unique, text-driven gifs for personal or professional use, enhancing digital communication with bespoke, eye-catching animations.
Lastly, the potential for educational applications, particularly for children, is immense. A discovery app powered by Stable Diffusion could generate images based on a child’s input, aiding in learning and exploration. Such an application could become an invaluable tool in interactive and visual learning.
By setting up Stable Diffusion locally, the possibilities for creative and practical applications are virtually limitless, offering bespoke solutions to a wide array of needs and industries.
Downloading and Configuring Stable Diffusion
In the realm of AI-driven image generation, downloading and configuring Stable Diffusion locally opens a plethora of optimization avenues. Here are advanced techniques that not only enhance the performance of Stable Diffusion but also tailor its functionality to specific user needs.
Use xFormers
xFormers, a transformer library developed by Meta AI, is pivotal in optimizing cross-attention operations in Stable Diffusion. This method reduces memory usage and boosts image generation speeds dramatically. In the Automatic1111 WebUI, enabling xFormers under the cross-attention optimization technique can lead to significant improvements in image generation speeds.
Use Smaller Image Dimensions
For those with less powerful GPUs, using smaller image dimensions can greatly speed up the image generation process. While this may limit the resolution of generated images, it’s an effective way to avoid memory errors and slow processing times. Upscaling techniques within Stable Diffusion can then be employed to enhance the image quality.
Use Token Merging
Token Merging is an advanced technique to speed up Stable Diffusion by reducing the number of tokens processed. This method combines inconsequential or redundant tokens, which can slightly improve image generation times. It’s recommended to use a low Token Merging value to avoid drastic changes in the output image.
Reduce Sampling Steps
Adjusting the sampling steps, which are the iterations Stable Diffusion goes through to generate an image, is another technique to enhance speed. Lowering the number of sampling steps can quicken image generation, but it’s crucial to find a balance to avoid compromising image quality.
Ensure GPU Utilization
Ensuring that Stable Diffusion is utilizing the GPU instead of the CPU is fundamental for optimal performance. In cases of misconfiguration or installation errors, the model may default to CPU usage, leading to slower generation speeds. Checking GPU usage in the Task Manager can confirm whether the GPU is being effectively utilized.
Upgrade/Downgrade GPU Drivers
The performance of Stable Diffusion can also be influenced by GPU drivers. Sometimes, upgrading or downgrading drivers, especially for Nvidia GPUs, can have a significant impact on the speed of image generation. Experimenting with different driver versions might reveal the best configuration for your specific hardware.
Switch To A Different Web UI
Different Web UIs for Stable Diffusion offer varying levels of optimization and performance. Switching from a less optimized UI like Automatic1111 to more efficient ones such as ComfyUI or InvokeAI can result in faster image generation and the ability to handle higher resolutions or more complex models.
Disable Unnecessary Apps & Services
Running Stable Diffusion alongside other resource-intensive apps or services can slow down the image generation process. Disabling unnecessary background apps and services can free up RAM and processing power, thereby improving the performance of Stable Diffusion, especially on systems with limited resources.
Reinstall Stable Diffusion
As a last resort, reinstalling Stable Diffusion can resolve any underlying issues that might be hampering its performance. A fresh installation ensures that the latest version is used and that all components are correctly configured and up-to-date.
Each of these techniques offers a way to optimize Stable Diffusion for faster, more efficient, and higher quality image generation, catering to the diverse needs and hardware capabilities of users.
Use cases with Stable Diffusion
Running Stable Diffusion locally is not just about generating AI images; it’s about harnessing this technology to create innovative applications that can transform various aspects of our digital life. When you set up Stable Diffusion on your own server, you unlock the potential to develop unique applications that leverage the power of AI for creative, educational, and practical purposes.
App for Generating AI Avatars
Imagine an application that lets users create lifelike avatars of themselves or others. This could revolutionize social media interactions, online gaming, and virtual reality experiences. By feeding in simple text descriptions or base images, users could generate detailed, personalized avatars that could be used across various digital platforms.
App for Generating NFT Collections
There’s a growing interest in the digital art world for unique, AI-generated artworks. With a local Stable Diffusion setup, developers can create applications that enable artists and photographers to generate and store collections of AI-created images as non-fungible tokens (NFTs). This opens up a new marketplace for digital art, where creators can sell unique, AI-generated pieces, adding a new dimension to the world of digital collectibles.
Chat Extension for Visual Communication
A chat extension that uses AI to visualize thoughts and ideas could change the way we communicate. Users could type in their thoughts, which the AI then converts into images, providing a visual representation of their ideas or feelings. This could be particularly useful in educational settings or as a tool for creative brainstorming sessions.
Automated Blog Cover Generator
For bloggers and content creators, an automated cover generator could be a game changer. This application would use the title and content of a blog post to generate a relevant and visually appealing cover image. Such an application would not only save time but also add a professional touch to blog posts, making them more engaging and shareable.
YouTube Video Creator
Stable Diffusion could be used to generate YouTube videos based on text prompts. This application would be incredibly useful for creating educational content, storytelling, or entertainment videos, providing creators with a tool to visualize their scripts or ideas in video format. The application could automatically generate scenes or animations based on the given text, making content creation more accessible and efficient.
GIF Generator
A GIF generator app using Stable Diffusion could provide endless fun and creativity. Users could input text to create customized GIFs, which could be used in social media, digital marketing, or just for personal enjoyment. This would add a new layer of personalization to digital communications, allowing users to express themselves in unique and creative ways.
Discovery App for Children
Finally, an educational app for children that uses Stable Diffusion to generate images based on their text input could be a powerful learning tool. Such an app would help children explore and discover new concepts and ideas, fostering creativity and curiosity. It could be a valuable resource in classrooms or at home, providing a fun and interactive way for children to learn about the world.
By running Stable Diffusion locally, developers can tap into these diverse applications, each catering to different needs and interests, and all powered by the transformative capabilities of AI.
Conclusion and Further Resources
As we’ve explored the diverse applications and technical nuances of Stable Diffusion, it’s evident that this AI model is not just a tool for image generation but a gateway to a myriad of creative and practical possibilities. The world of AI and machine learning is constantly evolving, with new advancements and applications emerging regularly. The future of AI-driven image generation, particularly with models like Stable Diffusion, holds vast potential.
Emerging Trends in AI Image Generation
Innovative Techniques:
- Stable Diffusion Img2Img: This technique has revolutionized the field of AI image generation. Unlike traditional models, Stable Diffusion Img2Img can create images that are not only of high quality but also consistent and stable, meaning they are robust to small changes in input. This stability is crucial in fields like digital art and game development.
Key Components:
- Noise Schedule: A critical component dictating the amount and type of noise introduced at each step of the diffusion process. It ensures smooth transitions from original data to random noise and back, contributing to the high-quality images.
- Denoising Score Matching: This technique estimates the probability distribution of data, crucial for accurately reversing the diffusion process and transforming random noise into recognizable images.
- Diffusion-based Image Generation: This process starts with the original image data, transforming it into random noise and then back into a new image. This method is flexible and produces high-quality, realistic images.
Practical Applications:
- Art and Creative Image Generation: Artists and designers can leverage Stable Diffusion Img2Img to generate unique and diverse artistic renditions.
- Data Augmentation for Machine Learning: This model can expand datasets in machine learning by generating new images, enhancing the robustness and accuracy of models.
- Medical Imaging and Diagnostics: It can play a significant role in the medical field by generating scans or images for disease detection and diagnostics.
- Video Game Development: Stable Diffusion Img2Img can be used to generate realistic landscapes, characters, and textures in video games and virtual environments.
As we move forward into an era where AI intertwines more seamlessly with our daily lives and professional fields, staying updated with the latest advancements in AI image generation is crucial. For further exploration and in-depth understanding, numerous resources are available online, including research papers, tutorials, and forums dedicated to AI and machine learning. Engaging with these resources will not only broaden your knowledge but also inspire new ways to utilize AI models like Stable Diffusion in your projects and endeavors.
Newsletter
You Do Not Want to Miss Out!
Step into the Future of Model Deployment. Join Us and Stay Ahead of the Curve!