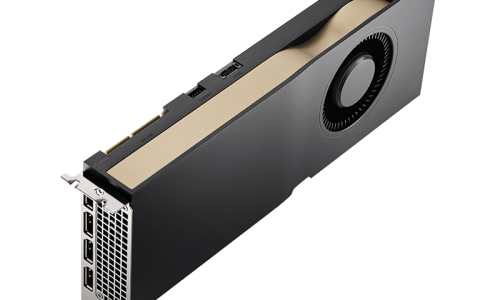
Performance and Speed of the Nvidia H100
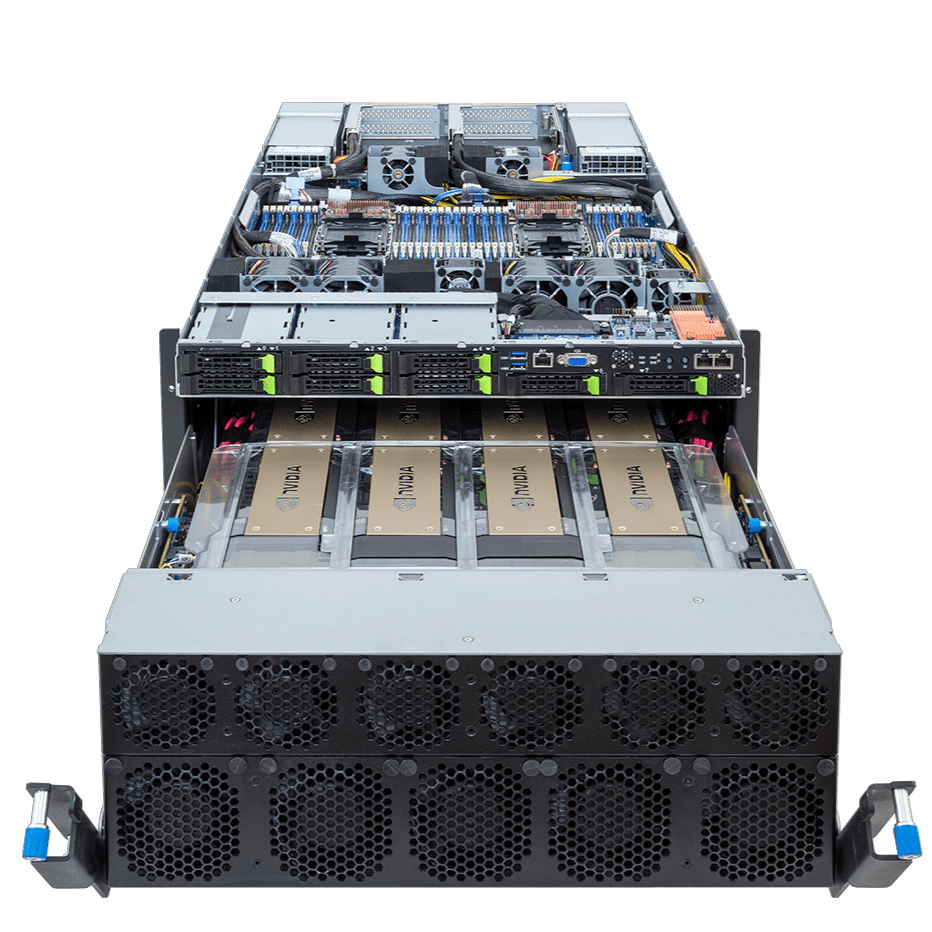
Introduction to Nvidia H100
The Nvidia H100 represents a paradigm shift in the realm of GPU server platforms. As the latest offering in Nvidia’s esteemed lineup, it is powered by the groundbreaking Nvidia Hopper Architecture, a leap forward in GPU technology. This architecture endows the H100 with unparalleled performance and scalability, especially critical in the fields of AI and High-Performance Computing (HPC). Compared to its predecessor, the A100, the H100 stands out in its ability to efficiently handle extensive and complex workloads. This includes, but is not limited to, generative AI, natural language processing, and deep learning recommendation models, all of which are increasingly pivotal in today’s technology-driven world.
Moreover, the H100 is not just a GPU; it is a full-stack solution that integrates a comprehensive range of capabilities. From networking to computing, it is designed to meet the diverse needs of modern data centers. This integration is particularly significant, as it enables the H100 to cater to a wide array of computational demands while maintaining efficiency and scalability. This versatility is essential in an era where the boundaries of digital computation are constantly being pushed.
Another notable feature of the H100 is its enhanced connectivity. With the NVIDIA NVLink Switch System, it is possible to connect up to 256 H100 GPUs, thereby accelerating exascale workloads. This capability is vital for tackling the most demanding computational tasks, ranging from intricate data analytics to cutting-edge AI applications.
In terms of technological innovation, the H100 boasts several new features that set it apart. These include a fourth-generation Tensor Core, a new Tensor Memory Accelerator unit, an advanced CUDA cluster capability, and HBM3 dynamic random-access memory. Each of these components plays a critical role in enhancing the H100’s performance, making it suitable for a wide range of high-performance computing, AI, and data analytics workloads in data centers.
Furthermore, the H100’s prowess is exemplified in its performance with large-scale AI models, such as GPT-3 175B, where it can deliver up to 12 times higher performance compared to previous generations. This capability is particularly beneficial for inference and mainstream training workloads, underscoring the H100’s suitability for current and emerging AI challenges.
In addition to its computational capabilities, the H100 also emphasizes security and trustworthiness. It supports confidential computing, which involves protecting data in use by performing computations in a hardware-based, attested trusted execution environment (TEE). This aspect of the H100 ensures that it is not only powerful but also secure, aligning with the increasing focus on data security in computing environments.
The Power of H100 in AI and Machine Learning
The Nvidia H100 has set a new standard in the realm of AI and Machine Learning, particularly in handling large language models (LLMs) that are central to generative AI. This advancement is not just a step but a leap forward, as evidenced by the H100’s performance in the MLPerf training benchmarks. In these highly-regarded industry-standard tests, the H100 has demonstrated exceptional prowess, setting new records across all eight tests. This includes its impressive capabilities in generative AI, a field that is rapidly evolving and increasingly significant in various sectors ranging from healthcare to finance.
A key factor behind the H100’s groundbreaking performance is its new engine, combined with the NVIDIA Hopper FP8 Tensor Cores. This combination allows the H100 to achieve up to nine times faster AI training and an astounding thirty times faster AI inference speedups on large language models compared to its predecessor, the A100. This kind of performance is not just an incremental improvement; it represents a transformative change in the way AI and machine learning workloads can be executed, offering opportunities for more complex and intricate AI models and applications.
In the context of large-scale AI deployments, the H100’s capabilities become even more pronounced. For instance, in the MLPerf Training v3.0, up to 3,584 H100 Tensor Core GPUs were used to set a new at-scale record of just under 11 seconds for training the ResNet-50 v1.5 model. Such performance highlights not only the raw power of the H100 but also its scalability and efficiency in real-world applications. Moreover, an 8.4% improvement in per-accelerator performance, thanks to software optimizations, illustrates the continual advancements being made in the H100 ecosystem.
The prowess of the H100 in AI inference is equally noteworthy. When running in DGX H100 systems, these GPUs have delivered the highest performance in every test of AI inference, a critical function for running neural networks in production environments. The GPUs showed up to 54% performance gains from their debut, a testament to the constant enhancements through software optimizations.
One of the most striking examples of the H100’s capabilities is seen in its performance on the Megatron 530B model. Here, the H100 inference per-GPU throughput was up to 30 times higher than the A100, with a response latency of just one second. This performance not only showcases the H100 as the optimal platform for AI deployments but also highlights its potential to revolutionize low-latency applications, a critical factor in numerous real-time AI scenarios.
High-Performance Computing (HPC) Capabilities
In the echelons of high-performance computing (HPC), the Nvidia H100 stands as a colossus, redefining the frontiers of computational capabilities. This cutting-edge GPU, a jewel in the crown of Nvidia’s Hopper architecture, has been intricately designed to cater to the most demanding HPC, artificial intelligence (AI), and data analytics workloads prevalent in contemporary data centers. The H100’s architecture, characterized by its innovative Tensor Core technology and a host of other advanced features, offers an unprecedented blend of performance, scalability, and security for a wide range of data center applications.
Delving deeper into its architectural prowess, the H100 is tailored for optimal connectivity, a cornerstone in HPC applications. It achieves this through its compatibility with NVIDIA BlueField-3 DPUs, allowing for 400 Gb/s Ethernet or NDR 400 Gb/s InfiniBand networking acceleration. Such high-speed connectivity is vital for secure and efficient handling of HPC and AI workloads. The H100’s impressive memory bandwidth of 2 TB/s further facilitates accelerated communication at a data center scale, ensuring that even the most data-intensive tasks are managed with remarkable efficiency.
One of the key aspects of the H100’s performance in HPC is its ability to deliver supercomputing-class performance. This is made possible through several architectural innovations, including the fourth-generation Tensor Cores and a new Transformer Engine, which are pivotal in accelerating large language models (LLMs). Additionally, the latest NVLink technology incorporated into the H100 allows for high-bandwidth, low-latency communication between GPUs at a staggering rate of 900GB/sec. This feature is critical in multi-GPU setups where collaborative processing of complex computations is required, a common scenario in HPC applications.
Furthermore, the H100’s design reflects a deep understanding of the nuanced requirements of modern HPC environments. Its capabilities extend beyond sheer computational power to encompass aspects of energy efficiency, space optimization, and ease of integration into existing infrastructure. This holistic approach positions the H100 as not just a powerful computing resource but also as a versatile and adaptable solution for a variety of HPC scenarios, ranging from scientific research to industrial simulations.
Real-World Applications and Case Studies
The Nvidia H100, far from being a mere technological upgrade, has emerged as a revolutionary force in AI and machine learning. It delivers up to 9 times faster AI training and 30 times faster AI inference in popular machine learning models compared to its predecessor, the A100. This substantial leap in performance is attributed to the new Transformer Engine, specifically designed to accelerate machine learning technologies. Such advancements are crucial in crafting the world’s largest and most complex ML models, marking a significant stride towards making AI applications mainstream.
Nvidia’s advancements extend to the server side, where businesses, assuming financial capability, can achieve supercomputer-class performance using the new DGX H100 servers. This capability democratizes access to powerful computing resources, previously the domain of well-funded research institutions or large corporations. The DGX H100 servers represent a paradigm shift in how businesses approach computational challenges, enabling them to harness supercomputing power for a range of applications.
A key development in this realm is the expansion of the proprietary NVLink interconnect. This innovation allows for the linking of individual server nodes, effectively creating a “data center-sized GPU.” This leap in connectivity and communication efficiency between GPUs enhances the capability to handle large-scale, complex computational tasks, a feature that is increasingly important in today’s data-intensive world.
The H100 also brings advancements in GPU virtualization. Each H100 can be divided into up to seven isolated instances, making it the first multi-instance GPU with native support for Confidential Computing. This feature ensures the protection of data in use, not just during storage or transfer, which is vital in a world increasingly conscious of data security.
In terms of scalability, the Nvidia H100 demonstrates its prowess through the DGX POD and SuperPOD configurations. Combining nine DGX H100 systems in a single rack creates a DGX POD, capable of delivering AI-based services at scale. The DGX SuperPOD, linking together 32 DGX systems and 256 H100 GPUs, delivers one Exaflop of AI performance with FP8 precision, a feat previously reserved for the world’s fastest machines.
Finally, Nvidia plans to build Eos, potentially the world’s fastest AI supercomputer, using 18 SuperPODs or 4,608 H100 GPUs. This ambitious project highlights the transformative potential of the H100 in advancing AI and computing at a global scale.
The NVLink’s role in these systems is pivotal. It has evolved from a GPU interconnect into a versatile tool for chip-to-chip connectivity. Supporting up to 18 fourth-generation NVLink connections, the H100 offers a total bandwidth of 900 GB/s, approximately seven times more than PCIe Gen 5. This evolution in NVLink is integral to the H100’s ability to handle the vast data bandwidth required in modern computational tasks.
Technological Innovations Behind H100’s Performance
The Nvidia H100 Tensor Core GPU stands at the pinnacle of technological innovation, redefining the standards of performance, scalability, and security across various workloads. At the heart of this transformative power is the NVIDIA NVLink Switch System, which facilitates the connection of up to 256 H100 GPUs, propelling exascale workloads to new heights. This system is complemented by the dedicated Transformer Engine, specifically designed to handle trillion-parameter language models, catapulting the large language model processing capability by an astonishing 30X over the previous generation, thereby setting new benchmarks in conversational AI.
Further driving the H100’s exemplary performance are the fourth-generation Tensor Cores, coupled with a Transformer Engine that operates with FP8 precision. This combination offers up to four times faster training for GPT-3 models compared to prior generations. The incorporation of fourth-generation NVLink, facilitating 900 GB/s GPU-to-GPU interconnect, NDR Quantum-2 InfiniBand networking for enhanced GPU communication, and NVIDIA Magnum IO software, collectively enable efficient scalability from compact enterprise systems to expansive unified GPU clusters.
The H100 also marks significant advancements in AI inference, accelerating this process by up to 30X and delivering minimal latency. The fourth-generation Tensor Cores, supporting various precisions, optimize performance while ensuring accuracy for LLMs, thus reinforcing Nvidia’s leadership in the inference domain.
Nvidia’s H100 exemplifies performance gains that surpass the expectations set by Moore’s law, particularly evident in its AI and HPC capabilities. It triples the FLOPS of double-precision Tensor Cores, yielding 60 teraflops of FP64 computing. The H100’s new DPX instructions offer seven times the performance of its predecessor, A100, and 40 times the speed of CPUs in dynamic programming algorithms, crucial for applications like DNA sequence alignment.
In the realm of data analytics, H100-powered accelerated servers deliver 3 TB/s memory bandwidth per GPU and scalability with NVLink and NVSwitch, making them adept at managing massive datasets. This capability, combined with NVIDIA Quantum-2 InfiniBand and Magnum IO software, enables unprecedented performance and efficiency in handling extensive data workloads.
The second-generation Multi-Instance GPU (MIG) technology in H100 optimizes GPU utilization by partitioning it into up to seven separate instances. This feature, along with the support for confidential computing, makes the H100 ideal for multi-tenant cloud service provider environments, ensuring secure and efficient resource allocation.
The H100 is also the first accelerator to incorporate NVIDIA Confidential Computing, a feature of the NVIDIA Hopper architecture. This technology creates a hardware-based trusted execution environment, safeguarding the confidentiality and integrity of data and applications in use, thus offering a secure solution for compute-intensive workloads like AI and HPC.
In addition, the H100 CNX combines the H100’s power with the advanced networking capabilities of the NVIDIA ConnectX-7 SmartNIC. This unique platform delivers unparalleled performance for GPU-intensive workloads, including distributed AI training and 5G processing at the edge.
Lastly, the Hopper Tensor Core GPU will power the NVIDIA Grace Hopper CPU+GPU architecture, specifically designed for terabyte-scale accelerated computing. This architecture, leveraging the Arm architecture for flexibility, will offer up to 30X higher aggregate system memory bandwidth to the GPU compared to current fastest servers, heralding a new era of performance for large-model AI and HPC applications.
Energy Efficiency and Environmental Considerations
The Nvidia H100 represents not only a leap in computational power but also a significant stride towards more sustainable computing. Nvidia’s approach to accelerated computing, as embodied in the H100, is fundamentally linked to sustainability. This philosophy acknowledges that the path to driving innovation across various industries must concurrently aim to reduce environmental impact. By optimizing the efficiency of computing processes, the H100 exemplifies how technological advancements can coexist with ecological responsibility.
A testament to the H100’s energy efficiency is its performance in the Green500 list, a ranking of the world’s most energy-efficient supercomputers. Nvidia GPUs, including those based on the H100, power 23 of the top 30 systems in this list. Particularly noteworthy is the H100 GPU-based Henri system at the Flatiron Institute in New York, which delivers an impressive 65.09 gigaflops per watt, retaining the No. 1 spot for energy efficiency.
The impact of the H100 on energy efficiency extends beyond rankings. In practical applications, the use of the latest H100 GPUs results in an order-of-magnitude reduction in both system costs and energy usage. This efficiency is crucial in today’s world, where the demand for computing power is escalating, but so is the need for environmental consciousness and cost-effectiveness. The H100’s architecture enables significantly faster processing of complex simulations and data analyses with a much lower energy footprint compared to previous generations and CPU-based systems.
Furthermore, the H100’s design incorporates features that specifically target energy efficiency. For example, its advanced memory technologies and streamlined data processing capabilities reduce the overall power required for large-scale computations. This design philosophy ensures that while the H100 delivers exceptional performance, it does so with a keen awareness of its energy use and environmental impact.
Future Outlook and Conclusion
As we embark on the next wave of high-performance computing and AI, the Nvidia H100 is poised to play a pivotal role in driving technological advancements and applications. Nvidia’s unveiling at SC23 showcased how their hardware and software innovations are shaping a new class of AI supercomputers. These systems, encompassing memory-enhanced Hopper accelerators and the new Grace Hopper systems architecture, will significantly enhance performance and energy efficiency for generative AI, HPC, and hybrid quantum computing.
The future also promises a dramatic performance boost with systems like the Nvidia H200 Tensor Core GPUs, which offer an 18x performance increase over previous generations. These GPUs, equipped with up to 141GB of HBM3e memory, are designed to run large generative AI models like GPT-4 with unprecedented efficiency. Moreover, new server platforms that link multiple Nvidia Grace Hopper Superchips via NVLink interconnects are set to deliver colossal computing power, essential for tackling the most demanding AI and HPC tasks.
Nvidia’s contribution to supercomputing is also witnessing substantial growth. The company now delivers more than 2.5 exaflops of HPC performance across world-leading systems, a significant increase from previous figures. This includes the Microsoft Azure Eagle system, which employs H100 GPUs for 561 petaflops of performance, illustrating the H100’s critical role in powering next-generation supercomputers.
In practical applications, the H100 is already demonstrating its potential to revolutionize various industries. For instance, Siemens’ collaboration with Mercedes to analyze aerodynamics for electric vehicles saw a significant reduction in simulation time and energy consumption, thanks to the H100’s capabilities. Such applications highlight the H100’s ability to reduce costs and energy use while delivering superior performance.
Looking ahead to 2024, the AI landscape is expected to be transformed with over 200 exaflops of AI performance on Grace Hopper supercomputers. The JUPITER supercomputer in Germany, capable of delivering 93 exaflops for AI training and 1 exaflop for HPC applications, exemplifies this leap in capability, all while maintaining energy efficiency. Furthermore, the deployment of GH200 supercomputers globally, from the U.S. to the Middle East and Japan, signals a widespread adoption of these advanced technologies for a range of applications, from climate and weather predictions to drug discovery and quantum computing.
Newsletter
You Do Not Want to Miss Out!
Step into the Future of Model Deployment. Join Us and Stay Ahead of the Curve!