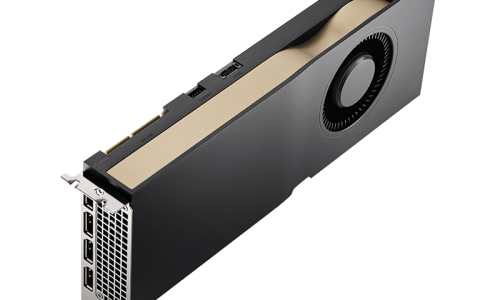
Scalability and Flexibility in GPU Rental Services
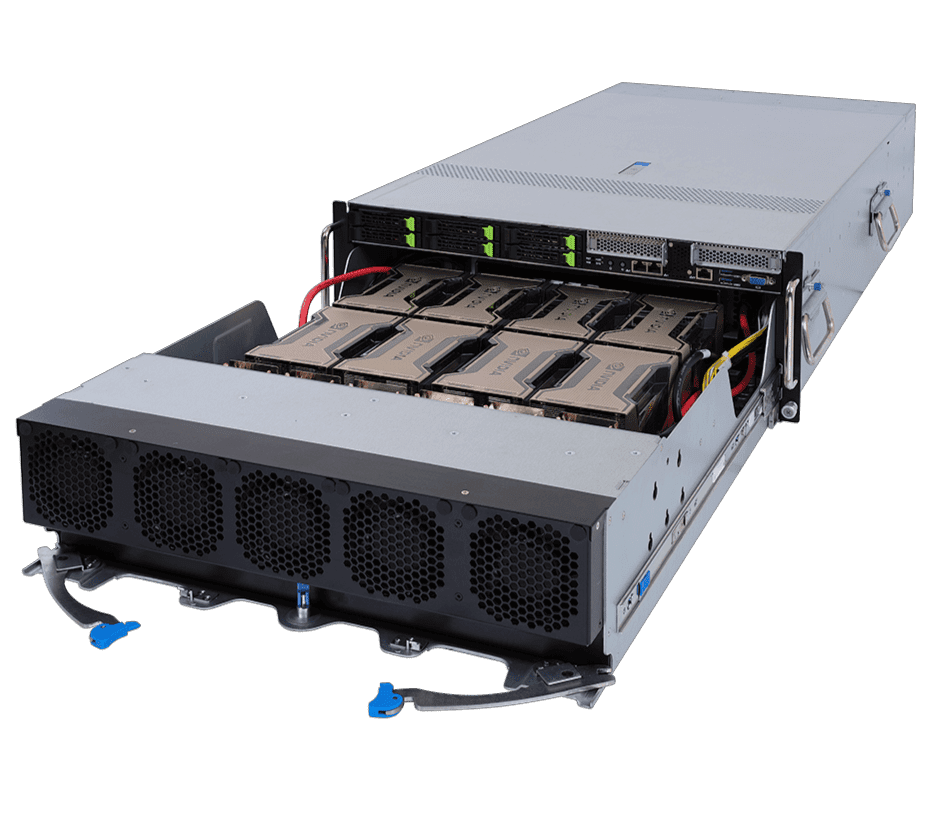
Introduction to GPU Rental Services
In the dynamic realm of cloud computing, the advent of GPU rental services marks a transformative phase, especially pertinent as we embrace 2023 and beyond. The essence of GPU-as-a-Service (GaaS) transcends conventional computing paradigms, offering an unparalleled combination of power and versatility for a diverse array of applications.
At the core, GaaS epitomizes the fusion of high-performance computing with accessibility. Its emergence is not just a technological evolution but a strategic imperative, catering to the ever-increasing computational demands across various sectors. The versatility of GPU rental services is evidenced in their widespread application, ranging from AI and machine learning advancements to the escalating computational needs in gaming and scientific research. Such diversity in application is a testament to the intrinsic adaptability and robustness of GPU-powered cloud environments.
The proliferation of GaaS is fundamentally redefining the computational landscape. It’s not merely about the processing power these services offer; it’s about the democratization of high-end computing resources. By leveling the playing field, GaaS enables entities, irrespective of their size, to harness the power of advanced GPU technologies. This democratization is pivotal in fostering innovation and accelerating developmental timelines across various industries.
Amidst this backdrop, 2023 stands as a critical juncture, witnessing a surge in the adoption of GPU rental services. The trends shaping this domain are multifaceted, encompassing increased adoption in traditional sectors and an expansion into novel realms like edge computing and AIaaS. This evolution is indicative of a broader shift towards more agile, scalable, and efficient computing solutions, a trend that is set to continue reshaping the technological and business landscapes alike.
As we delve into the intricacies of GPU rental services, it’s essential to acknowledge the broader implications of this technological shift. Beyond the technical capabilities, it’s about envisioning a future where computational barriers are diminished, fostering a new era of innovation and discovery.
The Rise of GPU-as-a-Service
The surge in GPU-as-a-Service (GaaS) encapsulates a significant shift in the technological ecosystem, particularly in the realm of high-performance computing. At the forefront of this transition is the booming market size of GaaS, which soared from USD 2.39 billion in 2022 to a projected USD 25.53 billion by 2030, a clear indication of its escalating relevance across various sectors.
The catalyst behind this remarkable growth lies in the burgeoning adoption of machine learning (ML) and AI-based applications. Industries ranging from finance to healthcare and automotive have increasingly integrated these applications, contributing to a substantial rise in the global AI adoption rate, as reported by the IBM Global AI Adoption Index 2022. This uptick in adoption underscores the demand for cost-effective and scalable computing resources, a niche perfectly filled by GaaS.
The versatility of GaaS extends beyond its computational prowess. During the COVID-19 pandemic, the acceleration in cloud-based services adoption highlighted the adaptability of GaaS. The pandemic-induced shift to cloud services enabled businesses to redirect funds towards R&D in ML and AI, further fueling the demand for cloud-based GPUs.
Innovation in the GaaS sector is also driven by the surge in deep learning, high-performance computing (HPC), and ML workloads. The escalating use of HPC technology by data scientists and engineers for processing large volumes of data and running analytics at a lower cost and time than traditional computing has been instrumental in this growth.
Yet, the journey of GaaS isn’t without its challenges. Data security remains a primary concern, as cloud storage and processing raise the risk of unauthorized access, data loss, and cyberattacks. However, the deployment models have evolved to address these concerns, with private and hybrid GPU clouds offering higher security levels.
In terms of enterprise adoption, large enterprises have been key players in utilizing GaaS solutions to overcome infrastructure maintenance challenges. Simultaneously, small and medium-sized enterprises are increasingly adapting to the digital environment to remain competitive.
The sectoral applications of GaaS are diverse, with the IT & telecommunication segment anticipated to dominate the market. The manufacturing segment is projected to experience the fastest growth, leveraging GPUaaS for managing large volumes of datasets for simulation and modeling jobs.
Regionally, North America leads in GaaS market share, driven by increased AI & ML adoption across various industries and robust government R&D funding. The Asia Pacific region shows the highest growth rate, with countries like India and China actively pursuing AI strategies. South America, the Middle East, and Africa are also embracing GaaS, with Brazil and GCC countries at the forefront of AI adoption in their respective regions.
Understanding Scalability in GaaS
Scalability stands as a cornerstone in the architecture of GPU-as-a-Service (GaaS), addressing the burgeoning computational demands across various sectors. The inherent design of GaaS platforms facilitates a seamless scaling of resources, allowing users to adjust their GPU requirements in real-time, contingent upon their computational needs. This dynamic scalability is a key differentiator from traditional on-premise GPU setups, where scaling often entails significant investments in additional hardware and complexities in managing data center space.
The transformational scalability of GaaS is deeply intertwined with the evolving nature of data-intensive tasks. Machine learning, deep learning, data processing, analytics, and high-performance computing (HPC) domains are particularly benefited from this. In these arenas, the ability to process vast amounts of data efficiently is paramount. GaaS platforms, with their parallel computing capabilities, not only enable but significantly accelerate these processes, rendering tasks like model training, data sorting, and financial modeling more efficient than ever.
Moreover, the scalability of GaaS is not confined to computational power alone. It extends to the realms of cost efficiency and resource allocation. The pay-per-use model intrinsic to GaaS ensures that organizations incur expenses only for the resources they utilize, thereby optimizing their expenditure and negating the need for hefty upfront investments typical of physical infrastructure setups. This financial scalability is especially crucial for small to medium-sized enterprises (SMEs) and startups, where budget constraints are often a key consideration.
In addition to scalability, GaaS platforms are noted for their ease of use and collaborative potential. User-friendly interfaces simplify the management of GPU resources, even for those with limited technical expertise. Furthermore, GaaS facilitates collaboration across geographical boundaries, enabling teams to share workloads and access the same datasets, thereby enhancing productivity and expediting project timelines.
As organizations increasingly migrate to cloud-based solutions, considerations around data security and compliance have become more critical. GaaS providers have responded by implementing robust security measures to safeguard sensitive information and ensure compliance with stringent industry standards such as GDPR or HIPAA.
In summary, the scalability of GaaS is multifaceted, encompassing computational power, cost efficiency, ease of use, collaboration, and security. This holistic scalability is pivotal in catering to the diverse and evolving needs of various industries, driving innovation and efficiency in an increasingly data-driven world.
The Flexibility Advantage
The flexibility inherent in GPU-as-a-Service (GaaS) platforms represents a pivotal shift in how computational resources are utilized and managed. This flexibility extends across various dimensions, fundamentally altering the landscape of high-performance computing.
Enhanced Resource Management
GaaS platforms exemplify adaptability in resource management. They empower users to effortlessly adjust GPU resources according to fluctuating computational needs, circumventing the constraints of acquiring additional hardware or grappling with data center space limitations. This dynamic capability is particularly vital in scenarios with irregular volumes or time-sensitive processes, typical in industries like gaming and complex ETL operations.
Cost-Effective Hybrid Deployments
The flexibility of GaaS extends to economic aspects as well. By adopting a cloud-driven, hybrid deployment model, businesses can significantly reduce upfront costs related to purchasing and maintaining dedicated GPU hardware. The pay-as-you-go pricing model inherent in GaaS aligns with the principle of economic scalability, enabling businesses to optimize cost management while maintaining high performance and connectivity across diverse environments.
Versatility and Global Accessibility
GaaS platforms are not just about computational flexibility; they also offer versatility in application and global accessibility. By leveraging high-performance GPUs through cloud delivery, a wide range of applications, from AI and machine learning to data analytics, can be significantly accelerated. This versatility allows users to run diverse workloads without needing specialized hardware setups. Additionally, cloud-based GPUs provide global accessibility, enabling geographically dispersed teams to collaborate effectively and facilitating remote work scenarios.
Addressing Infrastructure Challenges
The adoption of GaaS is a strategic response to the challenges in deploying and maintaining graphics-intensive infrastructure. It represents a shift from traditional GPU models that require substantial investments in physical machines and server instances. The managed GPU solutions in GaaS alleviate the need for massive servers and workstations within office premises, offering a streamlined approach that combines best-in-class infrastructure with advanced capabilities like NVIDIA H100.
Reducing Latency for Real-time Performance
Latency is a critical factor in high-end computing applications, where real-time performance is often a necessity. GaaS platforms are engineered to minimize latency, thereby enhancing application response and overall performance. This aspect is crucial in business-critical processes like voice modulation and retail customer analytics, where even slight delays can impact outcomes.
Simplified Coding and Management
The advent of GaaS has simplified the complexities associated with GPU processing. Coding for GPU applications is a specialized skill, and the managed solutions in GaaS provide an optimized platform that empowers multiple users to leverage the power of GPUs efficiently. This simplification is pivotal for businesses seeking to utilize advanced computing applications without the need for extensive in-house expertise.
In summary, the flexibility advantage of GaaS is multi-dimensional, encompassing resource management, cost efficiency, versatility, infrastructure simplification, latency reduction, and ease of coding and management. This comprehensive flexibility is reshaping the approach towards high-performance computing, making it more accessible and efficient for a wide range of industries and applications.
Latency and Performance
The integration of cloud-based GPU services in high-performance computing tasks brings into focus the critical aspect of network latency and its impact on overall performance. While GaaS platforms are engineered for efficiency and speed, the inherent nature of cloud services introduces latency challenges, particularly when compared to on-premise solutions with direct access to hardware resources.
Minimizing Network Hops
One effective strategy for mitigating latency in cloud-based GPU services is to minimize the network hops. The distance between the service requestor and the cloud-based processing service can add multiple latency-contributing hops. Optimizing the number of hops and processing requests at the closest possible point in the cloud provider’s network is crucial. Techniques like caching, direct routes, and geo-based routing are employed to reduce backend processing latency. Moreover, advancements in technologies such as 5G and edge computing play a pivotal role in reducing the number of hops and influencing the entire request journey, thus significantly minimizing latency.
Designing Scalable and Elastic Infrastructure
Addressing latency in GaaS also involves designing a horizontally scalable and elastic infrastructure. This approach ensures that resources can dynamically scale to accommodate fluctuating traffic, thereby providing consistent latency. Cloud architects often deploy auto-scaling groups, load balancers, and caching mechanisms to mitigate latency. By distributing the load among instances and reducing contention at individual servers, these constructs help maintain low latency, even as traffic grows. The scalable resources, enhanced by 5G’s data throughput capabilities and edge processing, play a vital role in reducing traffic to backend services, thus mitigating latency.
Optimizing Resource Allocation
Another effective approach is to identify bottlenecks and delegate requests to location and application-aware optimized resources. By directing the load to resources best suited for handling specific requests, processing performance improves, and latency is reduced. This method includes employing geo-based routing and request type-specific instances, and leveraging GPU compute resources for specific workloads like video analytics and machine learning, which can significantly speed up processing and limit latency.
Implementing Caching and Infrastructure Proximity
Caching is a critical factor in avoiding contention and minimizing latency. By caching the output of commonly used requests closer to the end user, the backend services need to process fewer requests, thereby reducing latency. The use of Content Delivery Networks (CDNs) and API caching are examples of caching strategies that help lower latency for data asset requests. Additionally, ensuring the closeness of dependent infrastructure through strategic location and connectivity is vital. By keeping interdependent resources closer to each other and optimizing network routing, the contribution to increased latency can be contained.
Traffic Segmentation and Policy Definition
Understanding traffic segmentation and defining policies for prioritization and processing are essential in reducing latency. Different types of traffic require different handling and prioritization strategies. Implementing geolocation-based routing and latency-based routing policies can help provide lower latency, as these policies enable processing by resources closer to the user. Network slicing is another technique that allocates capacity to prioritized traffic, maintaining quality of service even in the last mile transport, which is critical in maintaining latency, especially in high-contention scenarios.
In summary, addressing latency in GPU cloud services involves a multi-faceted approach, encompassing network optimization, scalable infrastructure design, bottleneck identification, caching strategies, infrastructure proximity, and traffic segmentation. These strategies collectively enhance the performance of cloud-based GPU services, ensuring that they meet the high-performance demands of various computational tasks.
Evaluating GPU Performance in Cloud Services
In the evolving landscape of cloud computing, particularly for GPU-as-a-Service (GaaS), evaluating GPU performance is crucial for ensuring optimal utilization of these resources. As businesses increasingly turn to AI, machine learning, and big data analytics, the demand for robust and efficient GPU resources in the cloud grows. However, not all workloads are suitable for GPU instances, and understanding the right context for their use is key to maximizing their potential.
Choosing the Right Instances for Specific Workloads
When considering GPU cloud servers for intensive tasks such as deep learning or database querying, selecting the right type of instance is critical. Dedicated instances are preferred over shared ones for such high-performance computing needs. These instances offer greater control and customization, allowing users to fine-tune their environment for optimal performance. Customizing instances with different types of GPUs optimized for specific workloads, like machine learning or graphics processing, ensures that the GPU resources are used most effectively.
Optimizing Memory Allocation
Proper memory allocation is vital for the efficient operation of GPU cloud servers. Incorrect allocation can lead to system sluggishness or, conversely, an inability to run certain tasks due to insufficient resources. It’s important to determine the precise memory requirements of applications to maximize GPU cloud server performance and minimize potential bottlenecks caused by inefficient resource allocation.
Regular Updates and System Maintenance
Keeping GPU cloud servers updated with the latest software and firmware versions is essential. Regular updates prevent bugs and ensure compatibility between the server’s hardware and software, as well as with other connected systems. This maintenance is crucial to avoid service disruptions or outages. Regular checks by IT teams on all installed applications are necessary to maintain the system’s performance at peak levels.
Continuous Monitoring and Tuning
Monitoring and tuning the performance of GPU cloud servers are essential practices. Tools like cAdvisor or system health monitors help in tracking the overall performance of the system and identifying potential problems like resource contention or hardware issues. Monitoring helps in identifying bottlenecks within the system, which can be caused by various factors such as inefficient resource allocation, outdated software, or hardware incompatibilities.
Addressing Resource Contention
Resource contention, where different processes compete for resources such as memory, disk space, and CPU cycles, can cause significant performance issues on GPU cloud servers, including decreased speeds and responsiveness. Regular system checks and optimization efforts by IT teams are critical in identifying and resolving these issues to ensure optimal performance.
In conclusion, evaluating GPU performance in cloud services involves a comprehensive approach, encompassing the selection of appropriate instances, memory optimization, regular system updates and maintenance, continuous monitoring and tuning, and addressing resource contention. Adhering to these best practices ensures that organizations can fully leverage the potential of GPU cloud servers, thereby deriving maximum value from their technology infrastructure investments.
Cost Considerations in GPU Rental
When it comes to the financial aspects of renting GPU services in the cloud, various factors come into play, influencing both the cost-efficiency and the overall expenditure associated with utilizing these services.
On-Demand and Subscription Models
GPU as a Service (GPUaaS) provides flexibility through its pricing models, which include on-demand and subscription services. On-demand services allow users to pay for GPU time on an hourly basis, providing a cost-effective solution for short-term, intensive tasks. Subscription services, on the other hand, offer access to GPUs for a set period, typically on a monthly or yearly basis, which can be more economical for long-term or continuous use cases. These models cater to the diverse needs of businesses and organizations requiring high-performance graphics processing for tasks like 3D rendering, video encoding, and gaming.
Rising Demand and Market Growth
The GPUaaS market, valued at approximately USD 3.0 billion in 2022, is expected to grow significantly, driven by the rising demand for high-performance GPUs. This demand is partly fueled by the growth of cryptocurrency mining operations. As digital currencies gain mainstream acceptance, the need for efficient GPUs for mining intensifies, propelling the GPUaaS market forward. The increasing requests from mining enterprises to utilize the computational capabilities of GPUs without the upfront costs of hardware ownership have expanded the revenue potential of GPUaaS providers and prompted them to innovate and optimize their offerings.
Financial Challenges and Considerations
Despite the benefits, there are financial challenges associated with GPUaaS. GPUs remain relatively expensive compared to other types of computing hardware, which can make GPUaaS services costly. Additionally, the cost of electricity required to run GPUs is a factor that organizations must consider. The scarcity of GPUs due to their high cost can also limit the availability of GPUaaS, posing a challenge for widespread adoption. Furthermore, the lack of standardization in GPUaaS offerings can make it difficult for consumers to find a provider that meets their specific needs.
In conclusion, while GPUaaS offers cost-efficient models and caters to the rising demand in various sectors, organizations must navigate the challenges related to costs, availability, and standardization to effectively leverage these services. Understanding the financial implications and selecting the right pricing model based on specific use cases are crucial steps in maximizing the benefits of GPU rental services.
Cost Considerations in GPU Cloud Services
The financial dynamics of GPU-as-a-Service (GaaS) are an essential aspect to consider, especially for businesses and organizations that heavily rely on high-performance graphics processing. Understanding the cost implications is crucial in deciding whether to integrate these services into their IT infrastructure.
Shifting from CapEx to OpEx
The GaaS model pivots the financial burden from capital expenditure (CapEx) to operational expenditure (OpEx). By opting for GaaS, organizations can avoid significant upfront investments in hardware, as well as the ongoing expenses associated with owning and maintaining physical infrastructure. This shift not only alleviates financial pressure but also offers a more flexible and scalable approach to resource allocation, aligning costs directly with usage and workload requirements.
The Impact of Cryptocurrency Mining
The rising demand from cryptocurrency miners has significantly impacted the GPUaaS market. With the growing popularity of digital currencies, there is an increased need for powerful and efficient GPUs for mining operations. This demand has expanded the revenue potential of the GPUaaS market and encouraged providers to optimize their offerings to cater specifically to the mining sector. The influx of mining enterprises seeking computational capabilities without the upfront hardware costs has been a notable driver in the expansion and diversification of the GPUaaS market.
Addressing Cost Challenges
Despite the advantages, there are notable cost challenges in the GPUaaS model. The high cost of GPUs remains a significant factor, making GPUaaS potentially costly compared to other computing hardware. Additionally, the operational cost, including electricity to run these GPUs, is an important consideration. Moreover, the availability of GPUs and the lack of standardization in the service provision pose further challenges, potentially affecting the overall cost-effectiveness and accessibility of GPUaaS solutions.
In conclusion, while GaaS offers a flexible and scalable solution for high-performance computing needs, it is imperative for businesses to carefully assess the financial implications, including both the potential benefits and the challenges, to make informed decisions about integrating GPU cloud services into their operations.
Newsletter
You Do Not Want to Miss Out!
Step into the Future of Model Deployment. Join Us and Stay Ahead of the Curve!