![The 4 Major Corporate Fears About AI [and How Arkane Crushes Them]](https://arkanecloud.com/wp-content/uploads/elementor/thumbs/image-fears-r83x02fmwkg0873grkx2f5jvdsy2k1b00h5jqz1vi0.png)
Text-to-Image Generation using AI
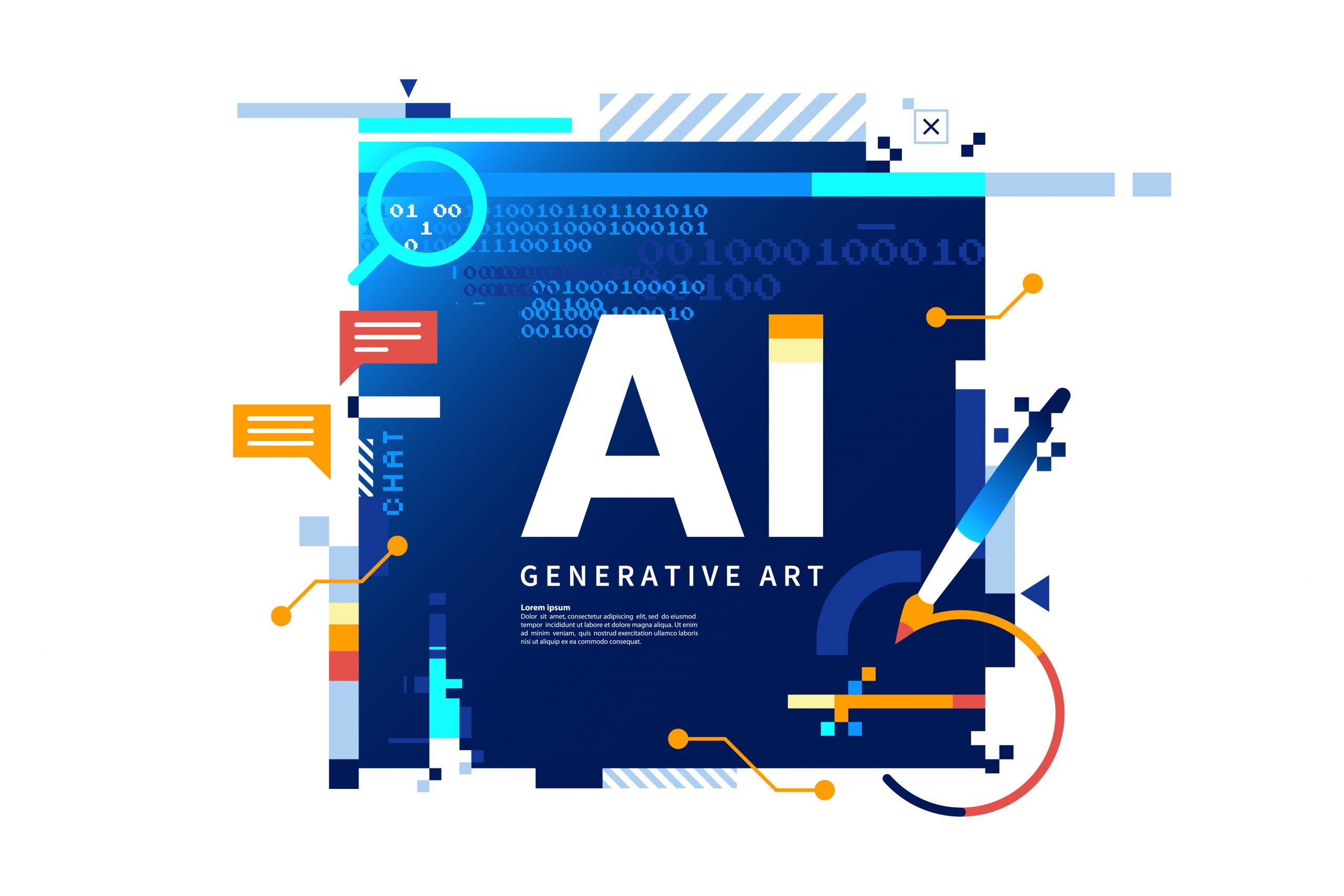
Introduction to AI-Driven Text-to-Image Generation
The advent of AI-driven text-to-image generation represents a significant leap in the realm of digital creativity. This technology, epitomized by models such as OpenAI’s DALL-E, translates textual descriptions into vivid, often startlingly precise visual representations. This capability has not only fascinated technology enthusiasts and professionals but has also captivated the general public, marking a rare instance where a complex AI innovation has permeated mainstream consciousness.
The genesis of DALL-E, a groundbreaking text-to-image model, traces back to OpenAI’s exploratory efforts in late 2021. Researchers, experimenting with the idea of converting brief text descriptions into images, unexpectedly stumbled upon a technological marvel that transcended their initial expectations. Sam Altman, OpenAI’s cofounder, acknowledged the immediacy of its impact, emphasizing that the model’s potential was apparent without the need for extensive internal debate or testing. This realization underlines the intuitive and transformative nature of this technology.
Following DALL-E, other significant contributions emerged in the AI text-to-image landscape. Google’s Imagen and Parti, along with Midjourney and Stability AI’s open-source model, Stable Diffusion, diversified the field, each bringing unique attributes and capabilities. These developments reflect a broader trend of rapid advancement in AI, a journey marked by both excitement and apprehension. The scope of these models extends beyond mere novelty, promising a reshaping of creative processes across various industries.
The rapid evolution of AI in this domain has led to an array of applications, with implications for numerous fields, including entertainment, marketing, and design. The transformative potential of AI text-to-image models lies in their ability to convert conceptual thoughts into tangible visuals at unprecedented speed. For professionals in creative fields, this represents a paradigm shift, offering a tool that dramatically accelerates the journey from idea to visual representation.
As we delve into the intricacies of AI-driven text-to-image generation, it’s crucial to understand the technology’s mechanics, its diverse applications, and the broader societal and ethical implications it entails. The unfolding narrative of AI in image generation is a story of technological marvel, creative liberation, and complex challenges, a narrative that continues to evolve and surprise at every turn.
The Mechanics of AI Image Generation
The process of AI-driven text-to-image generation is an intriguing blend of computational creativity and machine learning prowess. At its core, this technology is rooted in generative AI, a subset of machine learning focused on creating new data, rather than merely analyzing or predicting. Generative AI models, like those powering text-to-image generation, are trained to generate outputs that closely resemble the data they have been trained on. This is a significant departure from traditional AI, which typically involves making predictions based on input data.
The journey from simple generative models, like Markov chains used for next-word prediction in text, to the sophisticated architectures of modern text-to-image AI, highlights a remarkable evolution in AI’s complexity and capability. While early generative models were limited in their scope and depth, today’s AI systems, underpinned by large datasets and intricate algorithms, are capable of generating detailed and nuanced images. This leap in complexity is a testament to the rapid advancement in the field of machine learning and AI.
Key to this advancement are the deep-learning architectures that have emerged in recent years. Generative Adversarial Networks (GANs), introduced in 2014, exemplify this. A GAN consists of two models: a generator that creates images and a discriminator that evaluates their authenticity. This competitive dynamic between the two models drives the generation of increasingly realistic images. Similarly, diffusion models, which iteratively refine their output to produce data samples resembling their training set, have been pivotal in creating high-fidelity images. Stable Diffusion, a popular text-to-image generation system, is built on this diffusion model architecture.
Another landmark development in AI has been the introduction of transformer architectures in 2017. Transformers, used in large language models like ChatGPT, encode data (words, in the case of language processing) as tokens and create an ‘attention map’. This map delineates the relationship between different tokens, enabling the model to understand context and generate relevant text or images. The ability of transformers to manage and interpret extensive data sets is a cornerstone in the development of sophisticated text-to-image models.
The intricate interplay of these advanced architectures and large-scale data processing enables AI to perform the seemingly magical task of generating images from text. This process is not just a mechanical conversion but involves a deep understanding of language, context, and visual representation, resulting in the creation of images that are both relevant and aesthetically coherent.
Evolution of Text-to-Image AI
The evolution of text-to-image AI is a fascinating chronicle of technological advancement and creative exploration. It’s a journey that has taken us from the rudimentary beginnings to the sophisticated, almost magical capabilities we witness today.
In the initial phase of text-to-image generation, the technology was quite rudimentary. Early models produced images that were often pixelated, lacked detail, and appeared unrealistic. These limitations were a result of the nascent state of machine learning and deep learning techniques during this period. However, as these technologies evolved, there was a marked improvement in the quality of the generated images, transitioning from simplistic representations to more intricate and realistic outputs.
The first significant leap in text-to-image AI came with the introduction of deep learning. In the mid-2010s, advancements in deep neural networks enabled the development of more sophisticated text-to-image models. These models began combining a language model, which transforms input text into a latent representation, and a generative image model, which produces an image based on that representation. This synergy between language understanding and image generation was a pivotal moment in the field, leading to the creation of images that increasingly resembled human-created art and real photographs.
One notable early model in the text-to-image domain was alignDRAW, introduced in 2015. This model, developed by researchers from the University of Toronto, marked a significant step forward. While the images generated by alignDRAW were not photorealistic and were somewhat blurry, the model showcased an ability to generalize concepts not present in the training data, demonstrating that it was not merely replicating but was capable of creative interpretation of text inputs.
2016 saw another breakthrough with the application of Generative Adversarial Networks (GANs) in text-to-image generation. These models, trained on specific, domain-focused datasets, began producing visually plausible images. While still limited in detail and coherency, this represented a notable step towards more realistic image generation.
The field experienced a quantum leap with the advent of OpenAI’s DALL-E in January 2021. This transformer-based system was a watershed moment in text-to-image AI, capturing widespread public attention and setting new standards for image quality and complexity. The subsequent release of DALL-E 2 in April 2022 and Stability AI’s Stable Diffusion in August 2022 further pushed the boundaries, creating images that were more complex, detailed, and closer to the quality of human-generated art.
The journey of text-to-image AI is a testament to the rapid advancements in AI and machine learning. From simple, pixelated images to stunningly detailed and realistic artworks, this technology continues to evolve, reshaping our understanding of creativity and the role of AI in artistic expression.
Practical Applications and Use Cases of AI Text-to-Image Generation
The realm of AI-driven text-to-image generation extends far beyond mere artistic experimentation. This technology is rapidly becoming a vital tool in various practical applications, fundamentally altering how we approach numerous tasks and industries.
Revolutionizing Computer Vision
In computer vision, text-to-image models are pioneering new methods for improving visual recognition algorithms. By generating synthetic data from textual descriptions, these models enable the creation of diverse datasets. These datasets are instrumental in training and refining the performance of visual recognition algorithms, which is particularly valuable in scenarios where real-world data is limited or difficult to obtain. This application of synthetic data is proving to be a game-changer in enhancing the accuracy and robustness of computer vision systems.
Enhancing Training Data Quality
The generation of training data through text-to-image AI is an innovative approach that adds significant value. By creating various images from a single text prompt or altering prompts to introduce diversity, AI models can produce extensive datasets that are both varied and representative. This process, while not a complete replacement for real-world data, significantly augments existing datasets, especially in complex recognition cases where nuanced visual concepts are essential. The integration of text generation models like GPT-3 with text-to-image models further enriches the diversity and specificity of these synthetic datasets.
Real-World Applications: Food Classification
An intriguing example of practical application is found in food classification. In a study involving 15 different food labels, synthetic data generated by DALL-E mini was used alongside real images to train classification models. The results were noteworthy: the combination of synthetic and real data yielded an accuracy of 94%, surpassing the 90% accuracy achieved with real data alone. This demonstrates the substantial potential of synthetic data in enhancing the performance of machine learning models in real-world applications.
General Observations and Future Potential
The consensus is that synthetic data, generated by AI text-to-image models, holds immense potential in constructing robust machine learning models. When crafted with well-constructed prompts, this synthetic data achieves high quality, aiding significantly in model training for real-world applications. However, it’s important to note that using this data requires careful oversight, especially in production-level scenarios. As AI continues to evolve, the role of synthetic data in developing datasets is expected to become increasingly crucial, marking a new era in AI-driven solutions.
The practical applications of AI text-to-image generation highlight the technology’s transformative impact across various industries, from enhancing machine learning model accuracy to revolutionizing computer vision and beyond.
Understanding AI’s Creative Process
The process by which AI text-to-image models interpret and transform complex language into visual imagery is a blend of advanced machine learning techniques and natural language processing (NLP).
Natural Language Processing in AI Models
NLP plays a pivotal role in text-to-image AI platforms. It involves the interaction between computers and human language, where the AI uses NLP to analyze textual descriptions and extract relevant information. This information is then utilized to generate the corresponding images. NLP algorithms, trained on extensive datasets of human language, use statistical and machine learning techniques to recognize patterns and structures in language. This training allows them to grasp the nuances and complexities of human language, enabling the generation of accurate image descriptions from textual inputs.
Generative Adversarial Networks (GANs)
GANs are a type of machine learning model instrumental in generating new content, such as images or videos. They consist of two neural networks: a generator that creates images based on textual descriptions, and a discriminator that distinguishes between real and generated images. The continuous training and improvement of these networks result in the generation of high-quality, realistic images, which have a wide range of applications in various fields.
Transformer Models and Image Generation
Modern text-to-image models, like Imagen and Parti, build upon transformer models, which process words in relation to each other within a sentence. This is fundamental to representing text in these models. For instance, Imagen, a diffusion model, learns to convert a pattern of random dots into increasingly high-resolution images. Parti takes a different approach by converting a collection of images into a sequence of code entries based on the text prompt, effectively translating complex, lengthy prompts into high-quality images. Despite their sophistication, these models have limitations, such as difficulty in producing specific counts of objects or accurately placing them based on spatial descriptions. Addressing these limitations involves enhancing the models’ training material, data representation, and 3D awareness.
Broader Range of Descriptions
Recent advancements in machine learning have led to text-to-image models being trained on large image datasets with corresponding textual descriptions. This training has resulted in the production of higher quality images and a broader range of descriptions, marking major breakthroughs in the field. Models like OpenAI’s DALL-E 2 exemplify this progress, demonstrating the ability to create photorealistic images from a wide array of text descriptions.
The ability of AI to understand and interpret complex language to generate images is a testament to the intricate interplay of language processing, machine learning, and creative visualization. As these technologies continue to evolve, so too will the capabilities and applications of AI in the realm of text-to-image generation.
The Impact of AI on Creative Industries
The rise of AI in creative industries has been both transformative and controversial. AI’s ability to generate art, music, and other forms of entertainment has significantly changed the landscape of these fields.
Transformation in Creative Processes
AI is revolutionizing creative industries by offering new methods for idea generation and problem-solving. It has become a tool for optimizing existing processes, automating tedious tasks, and providing fresh perspectives. AI-assisted tools, now widely accessible to creative professionals, have introduced capabilities like generating visuals from images or text, AI music composition, and video editing with advanced effects. These tools have become integral to the creative toolkit, allowing professionals to work more efficiently and produce higher quality work. AI’s role in automating processes and pushing creative boundaries has opened up new avenues for exploring novel ideas and developing unique solutions.
Debate on Originality and Artistic Depth
AI-generated art has sparked heated debates over originality, authorship, and copyright. The ease with which non-artists can create artworks using text-to-image generators has led to a proliferation of AI-generated art, blurring the lines of traditional artistic skills. This rapid production and availability of AI art have raised concerns about the devaluation of human talent and the potential lack of creativity and artistic depth in AI-produced works.
Legal and Ethical Implications
The legal frameworks surrounding AI-generated art are still evolving, with varying interpretations depending on jurisdiction. Questions about who owns the copyright of AI-generated artwork—whether it’s the artist who created the prompt, the AI algorithm, or the developing company—have yet to be conclusively answered. This complexity is heightened when AI uses copyrighted works or existing images as a basis for generating new ones, leading to legal disputes and copyright infringement cases. Getty Images’ lawsuit against Stability AI is a notable example of these growing legal challenges.
Copyright Perspectives and Restrictions
Different countries have distinct laws regarding the copyright of AI-generated art. In the United States, for example, AI-generated art is viewed as the output of a machine and not eligible for copyright protection under federal standards, which require “human authorship” for a work to be considered for copyright. As a result, organizations and industries are increasingly cautious about using AI-generated art, with some opting to ban its use due to potential legal copyright issues. Major game development studios and scientific journals like Nature are among those that have imposed such bans.
The impact of AI on the creative industries is undeniable, bringing with it a host of new opportunities and challenges. While AI has enabled greater efficiency and novel creative expressions, it has also prompted a reevaluation of artistic originality, legal rights, and the ethical implications of machine-generated art.
The Future of AI in Image Generation
The future of AI in image generation promises a blend of enhanced capabilities, immersive experiences, and new ethical considerations.
Advancements in Image Quality and Realism
The ongoing evolution of text-to-image AI is set to further improve image quality, realism, and interpretability. Advances in multimodal learning, which involve the joint processing of text and images, are expected to lead to more sophisticated understanding and generation capabilities. This could mean even more lifelike and detailed images, pushing the boundaries of what AI can achieve in terms of visual accuracy and complexity.
Integration with Virtual and Augmented Reality
A significant future trend in text-to-image AI is its integration with virtual (VR) and augmented reality (AR). This integration is poised to revolutionize immersive experiences and digital storytelling. By combining the capabilities of text-to-image AI with VR and AR, new forms of interactive and immersive content can be created, offering unprecedented levels of engagement and creativity. This could transform fields like gaming, education, and entertainment, offering new ways to experience and interact with digital content.
Ethical Considerations and Responsible Development
As text-to-image AI becomes more pervasive, addressing ethical concerns and establishing responsible development and usage practices will be crucial. This involves creating regulations and guidelines to ensure transparency, fairness, and accountability in AI use. Intellectual property rights, data privacy, and the impact of AI on creative industries are some of the key areas that require careful navigation. Establishing a healthy and inclusive ecosystem for AI development and usage will be essential to harness its benefits while mitigating potential risks.
Enhancing Creative Processes for UI Design and Image Searches
Tools like Midjourney and OpenAI’s DALL-E are anticipated to bring transformative changes in fields such as app UI design and image searches. DALL-E’s potential in automating image generation offers a higher level of creativity for UI designers and app developers, streamlining the design process and enhancing user interfaces. Similarly, Google’s generative AI image creation tool highlights the evolving role of AI in transforming the way we conduct image searches, possibly leading to more intuitive and efficient search experiences.
The future of AI in image generation is not only about technological advancements but also about responsibly harnessing these innovations. It holds the promise of more detailed and realistic images, immersive AR and VR experiences, and new tools for creative industries, all while necessitating a mindful approach to ethical and societal implications.
Newsletter
You Do Not Want to Miss Out!
Step into the Future of Model Deployment. Join Us and Stay Ahead of the Curve!