![The 4 Major Corporate Fears About AI [and How Arkane Crushes Them]](https://arkanecloud.com/wp-content/uploads/elementor/thumbs/image-fears-r83x02fmwkg0873grkx2f5jvdsy2k1b00h5jqz1vi0.png)
What is a negative prompt stable diffusion
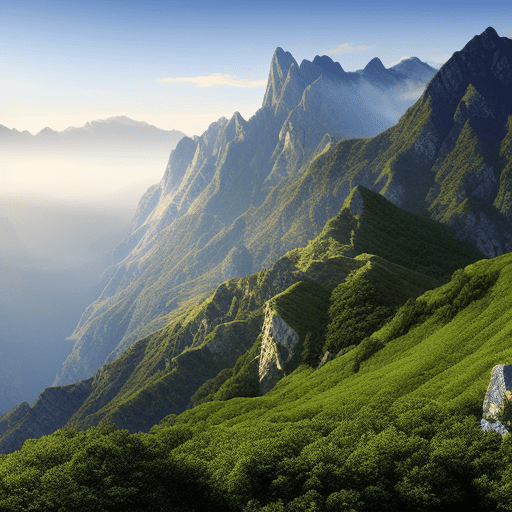
Introduction to Stable Diffusion and Negative Prompts
Stable Diffusion (SD) represents a quantum leap in the realm of artificial intelligence, particularly in image and language processing. At the heart of this innovation lies a community of over 200,000 members globally, dedicated to building the future of AI through open source research hubs. These hubs focus on developing cutting-edge models for diverse applications like image, language, audio, video, 3D, and biology, demonstrating the broad spectrum of SD’s influence.
The latest iteration, known as SDXL, exemplifies the rapid advancement in this field. It boasts a UNet backbone three times larger than its predecessors, a testament to its enhanced complexity and capability. The increase in model parameters, attributed to more attention blocks and an expanded cross-attention context, is facilitated by the integration of a second text encoder. This development underlines the commitment to designing novel conditioning schemes and adapting these models to various aspect ratios, showcasing the flexibility and scalability of SD technology.
Amidst this backdrop of innovation, negative prompts emerge as a pivotal concept in refining the output of AI models. These prompts, unlike traditional ones, guide AI by specifying what users do not want to see. This distinction is crucial for AI systems utilizing SD, as it empowers them to exclude unwanted elements, styles, or abnormalities, thus enhancing the accuracy and relevance of their outputs. Negative prompts serve as a fine-tuning mechanism, ensuring that the AI’s creations align more closely with user expectations and requirements. Their application ranges from image generation to language processing, making them a versatile tool in the AI toolkit.
The integration of negative prompts in SD reflects a nuanced understanding of AI’s capabilities and limitations. By defining what should be excluded, these prompts help to mitigate the generation of strange or irrelevant outputs, thereby enhancing the overall quality and precision of the AI’s work. In essence, negative prompts act as a filter, streamlining the AI’s focus and directing its computational resources towards producing results that are not only accurate but also contextually appropriate and user-specific.
In the realm of GPU server solutions, such as those provided by Arkane Cloud, the application of SD and negative prompts is particularly pertinent. As users seek to leverage these servers for AI generative tasks, machine learning, HPC, 3D rendering, and cloud gaming, the precision and adaptability offered by negative prompts become indispensable. They enable users to harness the full potential of GPU-powered AI, ensuring that the computational power is utilized efficiently and effectively, tailored to the specific needs and goals of each project.
This section sets the stage for a deeper exploration of negative prompts in stable diffusion, laying the foundation for understanding their significance in the broader context of AI advancements and applications.
Understanding Negative Prompts in Stable Diffusion
In the vanguard of AI technology, particularly in the context of GPU server solutions for tasks like AI generative art, machine learning, and cloud gaming, understanding the nuanced role of negative prompts in Stable Diffusion (SD) is essential. Unlike traditional prompts that guide AI towards a specific output, negative prompts serve as a boundary-setting mechanism. They instruct the AI on what to exclude from the output, acting as a creative constraint that enhances the precision and relevance of the results.
The transformative impact of negative prompts in AI art, especially in image generation, is a striking illustration of their potential. Artists and developers are leveraging these prompts to push the boundaries of creativity, producing artworks that blur the line between human and machine creativity. This innovative approach allows the AI to interpret contradictory inputs, transforming them into awe-inspiring visuals. Negative prompts have revolutionized the art world, enabling artists to explore new territories in image generation and photography.
In the practical application of negative prompts within AI systems, they operate by influencing the drafting process of image generation. By specifying characteristics or themes to avoid, they guide the AI to eliminate unwanted elements from the final product. This capability is particularly advantageous in scenarios where precision and adherence to specific artistic visions are critical. For instance, in creating a gaming environment or rendering a 3D model, employing negative prompts ensures that the output aligns perfectly with the intended design, free from any extraneous or undesired elements.
The use of negative prompts also plays a pivotal role in enhancing the diversity and creativity of AI-generated content. By directing the AI to avoid certain styles, subjects, or compositional elements, developers can craft more unique and visually striking outputs. This aspect is especially beneficial for cloud gaming and AI-driven entertainment, where uniqueness and visual appeal are paramount.
Furthermore, the implementation of negative prompts contributes to more stable and predictable AI outputs. By clearly defining what should not be included, these prompts help in fine-tuning the AI’s creative process, resulting in outputs that are cohesive and closely aligned with the user’s intent. For users of GPU server solutions, this means that their AI-powered projects are more likely to meet their specific requirements and standards, enhancing the overall efficiency and effectiveness of their computational resources.
In conclusion, negative prompts in Stable Diffusion represent a significant advancement in the field of AI, offering a new dimension of control and creativity. Their ability to refine AI outputs by exclusion rather than inclusion marks a paradigm shift in how we approach AI-generated content, particularly in industries reliant on precision and innovation, such as cloud computing, AI generative art, and gaming.
How Negative Prompts Work in Stable Diffusion
In the intricate world of Stable Diffusion (SD), negative prompts function as a sophisticated control mechanism, steering the AI away from undesired elements. This is in stark contrast to traditional methods where AI is directed towards generating specific outcomes. Negative prompts, therefore, serve as a strategic tool, particularly beneficial in GPU server solutions where precision and customization are paramount.
The process begins with the transformation of prompts into embedding vectors, which are then fed into the U-Net noise predictor within the SD model. Here, both positive and negative prompts are treated equally, each having 77 tokens, signifying their balanced importance in the AI’s decision-making process. This approach underscores the nuanced complexity of the SD algorithm, ensuring that it can cater to both inclusion and exclusion directives simultaneously.
The implementation of negative prompts is cleverly integrated into the sampler algorithms, which are responsible for the reverse diffusion process. In essence, negative prompts ‘hijack’ the unconditional sampling step, replacing an empty prompt that would typically generate random images. This strategic redirection enables the AI to actively avoid the elements described in the negative prompt, ensuring that the final output is free from undesired features or styles.
For instance, in GPU-based AI tasks like 3D rendering or complex simulations, the precision offered by negative prompts is invaluable. By instructing the AI on what not to include, users can fine-tune their models to generate outputs that align perfectly with their specific requirements, without the need for extensive manual adjustments or reiterations.
In conclusion, negative prompts in Stable Diffusion represent a breakthrough in AI content generation. They allow for a higher level of customization and precision, particularly vital in fields where detail and specificity are crucial. By understanding and utilizing these prompts effectively, users of GPU server solutions like Arkane Cloud can unlock new levels of efficiency and creativity in their AI-driven projects.
Practical Applications of Negative Prompts
The advent of negative prompts in AI, especially within the framework of Stable Diffusion (SD), has opened up a myriad of practical applications, transforming how technology interacts with creative processes. These prompts have become a vital tool in refining AI outputs, offering a level of precision and control essential for various tech-driven domains.
Refining AI-Generated Images
One of the primary applications of negative prompts lies in image generation. By specifying what not to include, users can significantly refine the output of AI art generators. This is particularly useful in scenarios where the AI might otherwise produce images that do not align with the user’s vision. Negative prompts act as a precise guide, ensuring the generated images meet specific aesthetic or thematic requirements.
Elimination of Unwanted Elements
In scenarios where specific elements or objects are undesirable, negative prompts play a crucial role. They enable users to directly instruct the AI to exclude particular items or features from the generated content. This feature is beneficial in customizing visual content for specific purposes, such as in advertising, where brand consistency and image are paramount.
Enhanced Functionality in Advanced Models
The evolution of Stable Diffusion models, from version 1.5 to the more advanced 2.x versions, showcases the improved functionality of negative prompts. The latter versions, equipped with OpenCLIP text encoders, offer more effective execution of negative prompts. This advancement signifies a leap in the AI’s ability to understand and implement user directives, making it a powerful tool in fields like cloud gaming and 3D modeling, where precision is key.
In conclusion, the practical applications of negative prompts in AI extend beyond mere image refinement. They offer a new level of control in AI-driven processes, essential for industries that rely on detailed and specific content generation. As technology continues to evolve, the role of negative prompts in shaping AI outputs will undoubtedly expand, offering even more sophisticated and tailored solutions for tech enthusiasts and professionals alike.
Newsletter
You Do Not Want to Miss Out!
Step into the Future of Model Deployment. Join Us and Stay Ahead of the Curve!