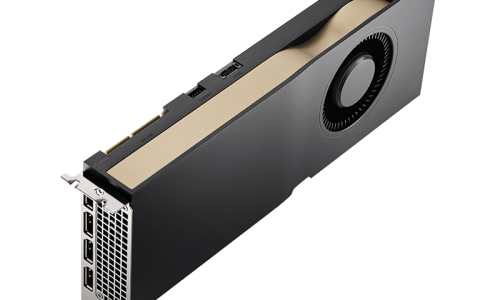
Introduction to Inference in Natural Language Processing (NLP)
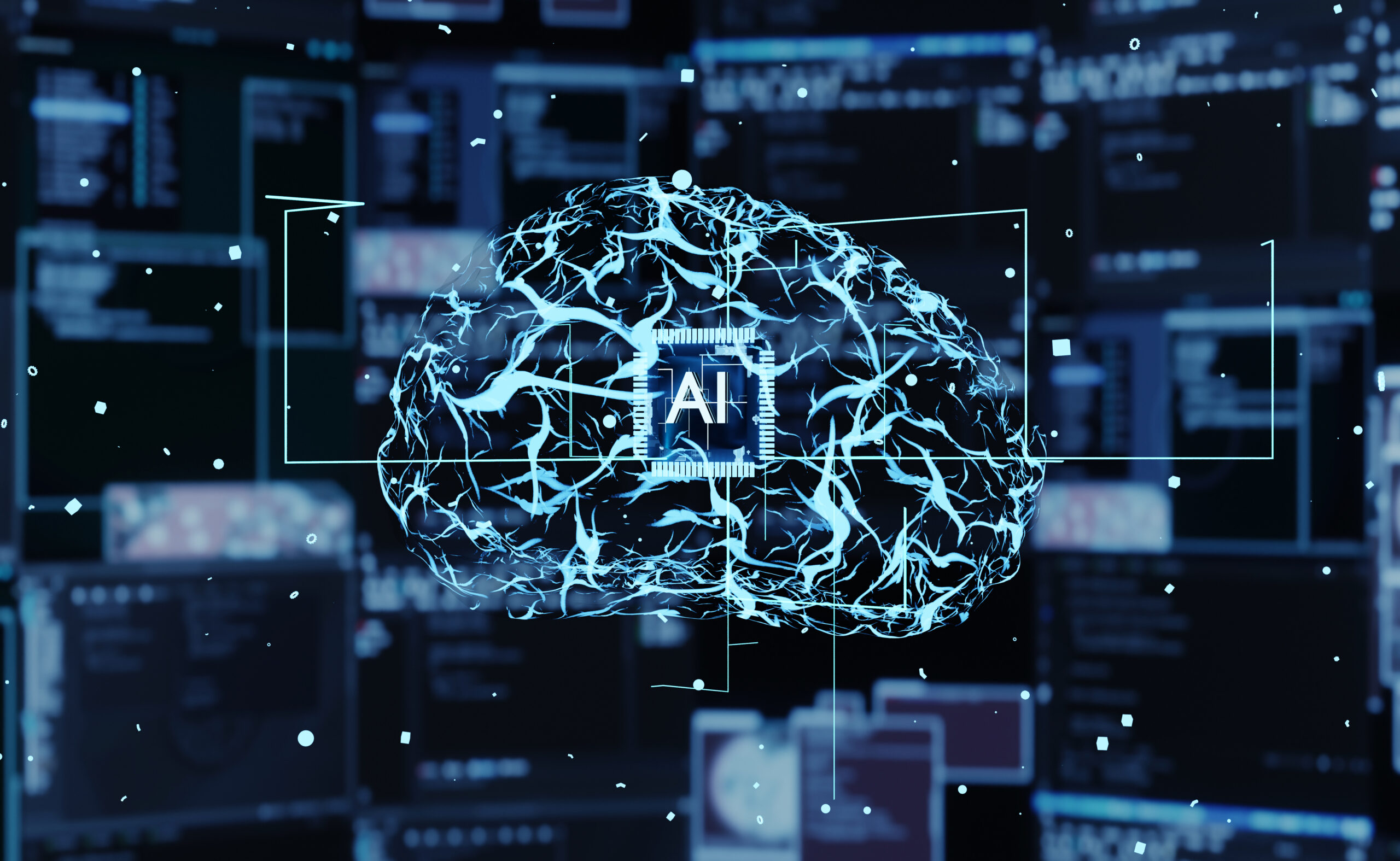
Introduction to Inference in Natural Language Processing (NLP)
In the dynamic world of NLP, inference is the silent powerhouse driving innovations from chatbots to complex data analysis. The year 2023 marks an era where the fusion of artificial intelligence (AI) and natural language processing is not just a scientific endeavor but a practical reality, touching every facet of digital interaction. With data being the new currency, the unstructured linguistic goldmine available online presents both challenges and opportunities.
The process of inference in NLP, where machines interpret and derive meaningful information from natural language, is akin to finding a needle in a haystack. It’s not just about understanding words but grasping nuances, emotions, and contexts. As such, the advancements in this field are not just incremental but revolutionary, pushing the boundaries of what machines can comprehend and how they respond.
Arkane Cloud, with its robust GPU server solutions, sits at the forefront of this revolution. Our servers are the bedrock upon which these sophisticated NLP models operate, providing the necessary computational power and speed. But, it’s not just about raw power. The evolution of NLP inference demands a delicate balance between speed, accuracy, and efficiency.
In recent years, there has been a significant shift towards optimizing large language models (LLMs) like GPT-3 and BERT. These models, known for their depth and complexity, are being fine-tuned to deliver more with less – less time, less data, and fewer computational resources. Techniques such as model distillation, which simplifies the models while retaining their capabilities, and adaptive approaches like prompt tuning, which customizes models for specific tasks without extensive retraining, are at the forefront of this transformation.
Furthermore, the trend of multimodal and multitasking models like DeepMind’s Gato signifies a move towards more versatile and robust AI systems. These systems can process and interpret various data types (text, images, audio) simultaneously, breaking the silos of single-modality processing.
Lastly, the synthesis models from text, exemplified by innovations in text-to-image models like Dall-E 2, are redefining the creative possibilities of AI. These models can generate high-resolution, contextually accurate visual content from textual descriptions, opening new avenues in digital art, design, and beyond.
In conclusion, NLP inference in 2023 is not just a study of language but a multifaceted exploration into how AI can seamlessly integrate into and enhance our digital interactions. Arkane Cloud’s GPU servers are more than just machines; they are the enablers of this linguistic and cognitive evolution.
Trends in NLP Inference
Virtual Assistants
2023 marks a significant leap in the evolution of virtual assistants, driven by advancements in natural language processing (NLP). These AI-powered assistants, embedded in various devices and applications, are increasingly becoming more adept at enhancing user accessibility and delivering information instantaneously. The critical factor behind their effectiveness lies in the precision of interpreting user queries without misinterpretation. NLP’s role is pivotal in refining these virtual assistants to minimize errors and ensure continuous, uninterrupted operation. Their utility extends beyond conventional roles, finding applications in assisting factory workers and facilitating academic research, a testament to their versatility and growing importance in diverse fields.
Sentiment Analysis
The digital age has ushered in an era where vast amounts of data in forms of audio, video, and text are generated daily. One of the challenges that emerged is the inability of traditional NLP models to discern sentiments in communication, such as distinguishing between positive, negative, or neutral expressions. This limitation becomes particularly evident in customer support scenarios, where understanding the customer’s emotional state is crucial. However, 2023 witnesses a transformative approach in NLP, with emerging models capable of comprehending the emotional and sentimental contexts within textual data. This breakthrough in NLP is significantly enhancing customer service experiences, fostering loyalty and retention through improved interaction quality.
Multilingual Language Models
In our linguistically diverse world, with over 7000 languages, the need for NLP models that transcend the predominance of the English language is more critical than ever. The traditional focus on English left many languages underserved. However, the current trend is shifting towards the development of multilingual language models, thanks to the availability of extensive training datasets in various languages. These advanced NLP models are adept at processing and understanding unstructured data across multiple languages, significantly enhancing data accessibility. This progress in NLP is not only a technological triumph but also a gateway for businesses to expand their reach and streamline translation workflows, thereby broadening their global footprint.
Innovations in NLP Inference
Named Entity Recognition (NER)
The recent advancements in NER, a critical component of NLP, revolve around deep learning architectures and the innovative use of large volumes of textual data. NER has evolved from simple linear models to more complex neural networks, significantly enhancing its ability to identify and classify entities such as names, organizations, and locations from vast amounts of unstructured text. This evolution is marked by the shift towards using sophisticated deep learning models and varied training methods that leverage both structured and unstructured data, enabling more accurate entity recognition and classification.
Language Transformers
Language transformers represent a significant leap in NLP. These transformers, unlike traditional models, utilize self-attention mechanisms, allowing them to understand the context and relationship between words in a sentence more effectively. This approach has drastically improved the efficiency and accuracy of NLP models in tasks such as translation, summarization, and question-answering. The unique architecture of language transformers, where the focus is on the relationship between all words in a text rather than sequential analysis, has paved the way for more nuanced and context-aware NLP applications.
Transfer Learning in NLP
Transfer learning has emerged as a game-changer in NLP, addressing the challenge of applying models trained on one task to another. This technique has allowed for more efficient use of resources, reducing the time and computational power needed to train NLP models. By transferring knowledge from one domain to another, NLP models can now be trained on a broader range of data, leading to more generalized and robust applications. This approach has significantly reduced the barriers to entry for developing sophisticated NLP applications, enabling smaller organizations and projects to leverage the power of advanced NLP without the need for extensive resources.
Utilizing Unlabeled Text and Embeddings
A noteworthy innovation in NLP is the effective use of unlabeled text and various embedding techniques. Unlabeled text, which forms the bulk of available data, is now being used to enhance the performance of NLP models. The integration of word and character embeddings, such as GloVe and character-level representations, has improved the ability of NLP systems to understand and process text data. These embeddings capture the nuances of language at both the word and character level, providing a richer understanding of language structure and meaning.
Application and Impact of NLP Inference
The field of NLP in 2023 has witnessed groundbreaking innovations, particularly in the areas of text summarization, semantic search, and reinforcement learning, driven by the continuous evolution of large language models (LLMs).
Text Summarization
Innovations in text summarization have significantly improved the ability of NLP models to distill and condense large volumes of text into coherent and concise summaries. This advancement not only saves time but also enhances the efficiency of information processing across various sectors. The development of models like PaLM-E exemplifies the integration of multimodal inputs into language models, thereby enriching the summarization process with contextual insights from various data types.
Semantic Search
Semantic search in NLP has transformed how we retrieve information, moving beyond keyword matching to understanding the intent and context of queries. This evolution has greatly improved the relevance and accuracy of search results, benefiting areas such as eCommerce, academic research, and enterprise knowledge management. The introduction of models like MathPrompter, which enhances LLMs’ performance in arithmetic reasoning, indicates the expanding capabilities of NLP models in specialized domains, further refining the semantic search process.
Reinforcement Learning in NLP
The incorporation of reinforcement learning in NLP marks a significant leap in model training and adaptability. This approach enables NLP models to learn from environmental feedback, optimizing their performance in various applications. Studies on in-context learning (ICL) reveal that larger models can adapt their learning based on context, showcasing the potential of reinforcement learning in enhancing NLP applications. This adaptive learning capability is crucial in scenarios where models encounter situations outside their initial training parameters, enabling continuous improvement and customization.
Future Prospects
The future of NLP inference appears incredibly promising, with new techniques like FlexGen demonstrating the potential to run LLMs efficiently on limited resources. This advancement is crucial for making NLP technology more accessible and scalable. Additionally, the exploration of multimodal large language models like Kosmos-1, which aligns perception with language models, indicates a move towards more integrated and comprehensive AI systems capable of reasoning beyond text, opening up new possibilities in NLP applications.
In summary, the advancements in NLP in 2023, from enhanced text summarization to innovative semantic search and adaptive reinforcement learning models, are redefining the landscape of natural language processing. These developments are not only technical milestones but also catalysts for broader applications of NLP in various domains, heralding a new era of intelligent and context-aware AI systems.
Newsletter
You Do Not Want to Miss Out!
Step into the Future of Model Deployment. Join Us and Stay Ahead of the Curve!