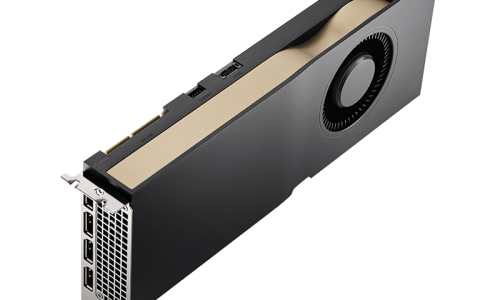
Text Generation for Chatbots and Virtual Assistants
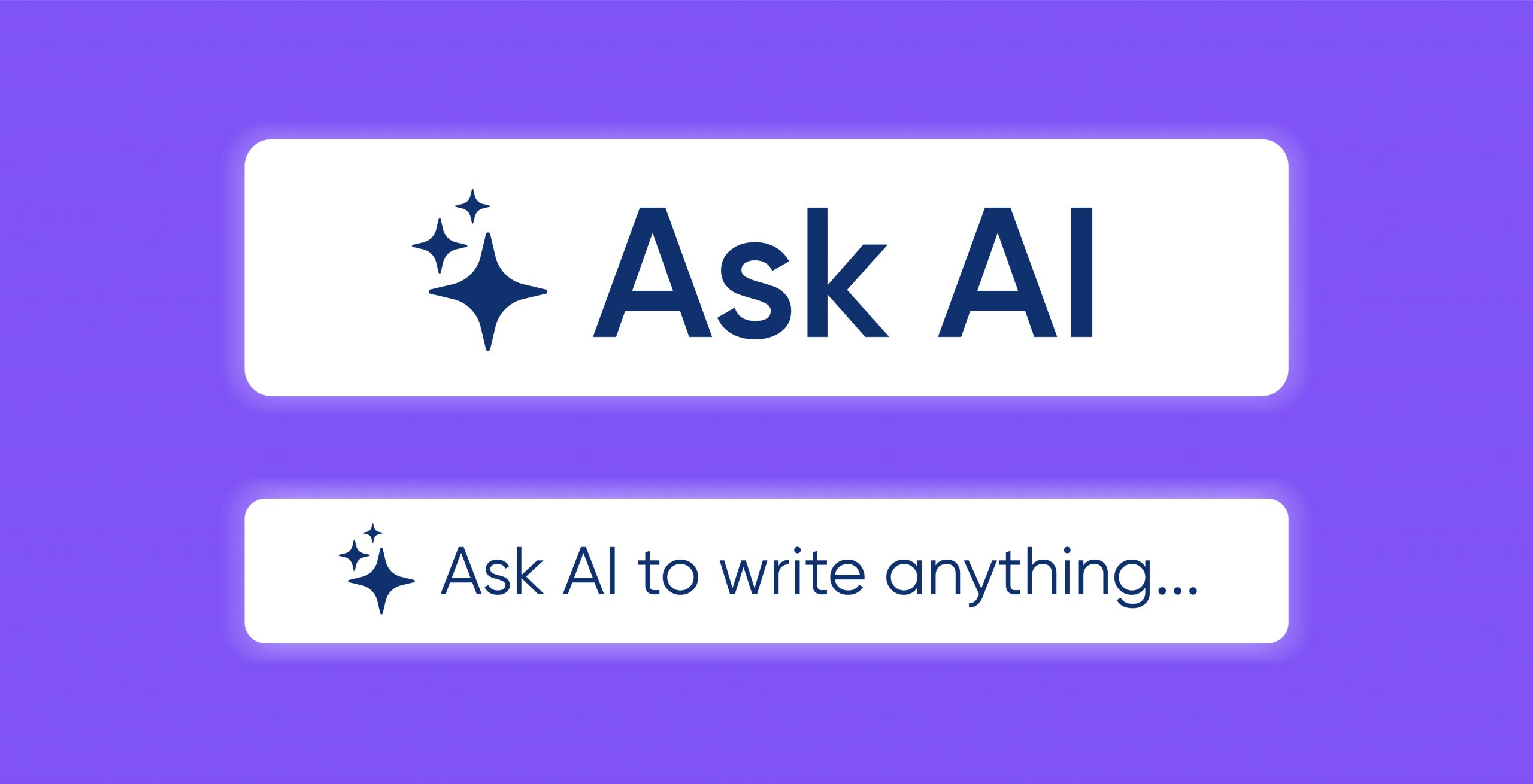
Understanding the Evolution of Conversational AI
Conversational AI has emerged as a pivotal component in the interaction between technology and humans. The recent surge in the adoption and development of Large Language Models (LLMs) like OpenAI’s GPT-3 has revolutionized this field. These models exhibit an exceptional understanding and generation of human-like text, shifting the paradigm in creating smarter chatbots and virtual assistants. This advancement marks a significant step away from rule-based chatbots towards more intuitive and natural interactions, propelling the technology to the forefront of artificial intelligence and human-computer interaction.
The transition from conventional rule-based chatbots to sophisticated LLMs symbolizes a monumental leap in the conversational AI landscape. Early chatbots, bound by predefined scripts and keyword-based responses, often resulted in rigid and unsatisfying user experiences. However, the advent of LLMs, leveraging deep learning and extensive internet text training, has enabled an understanding of context, syntax, and even sentiment, closely mirroring human conversation patterns.
This transformation is significant in the context of chatbots and virtual assistants, which are now increasingly capable of engaging in more dynamic and contextually aware dialogues. These AI-driven systems understand user queries and respond in a more human-like manner, making them crucial tools in various domains, including customer service, healthcare, and education. The role of conversational AI in enhancing human-computer interactions is becoming more profound, with these systems now capable of executing complex tasks, understanding nuanced language, and providing personalized responses.
In essence, the development of LLMs and their integration into conversational AI represents a leap towards more empathetic, intuitive, and efficient interactions between humans and machines. As these technologies continue to evolve, they are expected to become even more integral to our daily lives, reshaping how we interact with the digital world.
Navigating the Complexities of Conversational AI
Conversational AI, despite its advances, grapples with unique challenges that must be acknowledged and addressed to continue its evolution and widespread adoption. One of the most pivotal of these is the issue of bias within AI models. This stems primarily from the training data used to educate these models. With vast volumes of data being fed into these systems, the inherent biases present in this data are often overlooked, resulting in AI responses that may perpetuate stereotypes or exhibit partiality. Ensuring the creation of unbiased and inclusive training data is crucial but remains a largely unaddressed area.
Another significant challenge lies in the realm of misinformation propagation, especially with the widespread adoption of Large Language Models. While these models are adept at generating coherent text, ensuring the factual accuracy of this generated content is a complex task. This issue is amplified as AI becomes capable of generating large volumes of data without adequate fact-checking mechanisms.
Expanding conversational AI to cater to native languages presents its own set of difficulties. Not every language enjoys the same level of technical support and resources as some widely spoken languages. This disparity risks marginalizing certain linguistic groups who cannot leverage these advanced technologies for their specific needs.
In terms of customer and business adoption, conversational AI faces hurdles in engagement and investment. While customer interactions with chatbots and voice bots are increasing, there is still a segment of users who are distrustful or reluctant to engage with AI. Convincing businesses to invest in conversational AI is challenging, especially given the significant investment required and the current financial climate.
The market is also becoming increasingly crowded with conversational AI solutions, making differentiation a key challenge for vendors. It’s not enough to have advanced technology; vendors must demonstrate a holistic approach, including a robust implementation strategy, comprehensive educational content, and an accessible package tailored to customer needs.
One of the more subjective challenges is managing user expectations. With tools like ChatGPT raising the bar for conversational AI, users’ expectations for their interactions with all AI-driven platforms are also increasing. Meeting these heightened expectations requires continuous innovation and improvement in the AI technology.
These challenges, while formidable, are not insurmountable. They represent key areas for focused development and innovation in the field of conversational AI, paving the way for more advanced, equitable, and user-friendly AI interactions in the future.
Enhancing Conversational AI Through Strategic Interventions
Conversational AI is undergoing continuous transformation, propelled by advancements in technology and an expanding adoption rate within enterprises. This section will delve into strategies to enhance the naturalness and context understanding in conversational AI, focusing on training data improvement, context awareness advancement, and the integration of conversation mirroring techniques.
Training Data Enhancement
To augment the naturalness of conversational AI, there’s a growing emphasis on refining training data. This data serves as the bedrock of AI models, shaping their behavior and response patterns. OpenAI, for instance, is seeking partnerships to create more nuanced and conversational-style training datasets, focusing on data that encapsulates human intention across various languages and formats. This approach aims to produce AI models that better reflect the diverse and intricate nature of human conversations, thereby enhancing their naturalness and adaptability.
Advancing Context Awareness
A pivotal aspect of improving conversational AI is enhancing its context awareness. Contextual understanding is critical for generating meaningful and coherent responses. To address this, innovators are focusing on training conversational agents using domain-specific content, such as live agent logs, transcripts, and FAQs. However, the traditional methods of training, often manual and rule-based, are being reevaluated. Innovations in training are now geared towards automating and accelerating this process, with patents describing techniques like automatic generation of questions and responses, and deep learning-based unsupervised learning methods. These innovations aim to reduce the training time and improve the quality of responses, enabling conversational AI to handle more complex scenarios involving multiple commands or multitopic conversations effectively.
Conversation Mirroring Techniques
Conversation mirroring is another critical strategy for enhancing naturalness in conversational AI. This involves training AI models on extensive datasets of human conversations, allowing them to learn from real-world interactions. By modeling human conversational patterns, phrases, and responses, AI can generate more natural and contextually appropriate replies. The trend now is to move beyond traditional task-based conversations to more personal and autonomous interactions, with multimodality poised to play a significant role. This approach not only increases the naturalness of AI interactions but also enhances user engagement by requiring less input from users to resolve queries.
Collectively, these strategies represent a comprehensive approach to improving conversational AI. By focusing on the quality of training data, advancing context awareness, and incorporating conversation mirroring techniques, AI can become more adept at engaging in natural, contextually rich, and meaningful conversations with users.
The Evolutionary Leap of Large Language Models in Conversational AI
The evolution of Large Language Models (LLMs) in the field of Conversational AI represents a significant shift, particularly with the advent of models like ChatGPT by OpenAI. This evolution traces a path from early NLP models constrained by task-specific supervised learning to the transformative impact of ChatGPT, a chatbot optimized for dialogue and human-like interaction.
Pre-Transformer Era and the Advent of Transformer Architecture
Prior to 2017, NLP models were predominantly task-specific, trained under supervised learning protocols and limited to the tasks they were designed for. This changed dramatically with the introduction of the Transformer architecture in 2017. This innovation paved the way for groundbreaking models such as BERT and GPT, which utilized a semi-supervised approach combining unsupervised pre-training with supervised fine-tuning. This method allowed these models to apply pre-trained language representations to a wide range of downstream tasks, significantly enhancing their generalization capabilities.
The Emergence of GPT-3
A monumental leap in this evolutionary journey was the development of GPT-3. With its staggering 175 billion parameters, GPT-3 stands as a colossus in the realm of LLMs, dwarfing its predecessor GPT-2 and marking a significant advancement in the model’s capacity to generate contextually rich and nuanced text.
Ethical Alignment and User-Centric Approach
A major challenge in the evolution of LLMs is ensuring their alignment with human values and ethical principles. This issue of alignment underscores the complexity of developing AI models that not only perform efficiently but also adhere to ethical standards.
From InstructGPT to ChatGPT
The development of ChatGPT stemmed from InstructGPT, an LLM trained using Reinforcement Learning from Human Feedback (RLHF). This approach combined supervised learning to garner human feedback and reinforcement learning using human preferences as a reward signal. RLHF was instrumental in adapting GPT-3 to more accurately follow user instructions, leading to the creation of ChatGPT, optimized for dialog and user-centric interaction.
The evolution of LLMs, especially with models like ChatGPT, signifies a new era in Conversational AI, marked by advancements in natural language understanding, generation capabilities, and ethical alignment. These developments are reshaping the landscape of human-AI interaction, heralding a future of more intuitive, responsive, and ethically aligned conversational agents.
Empowering Conversational AI with Large Language Models
The incorporation of Large Language Models (LLMs) into conversational AI has significantly transformed the landscape of virtual interactions. Unlike their rule-based predecessors, LLMs such as OpenAI’s GPT-3 have revolutionized the way chatbots and virtual assistants understand and generate human-like text, leading to more natural, context-aware, and meaningful conversations.
Breakthroughs in Conversational AI
LLMs have brought about a paradigm shift in conversational AI. Traditional chatbots, confined to strict command-response mechanisms, often resulted in rigid and unsatisfactory interactions. They lacked the ability to adapt to the nuanced and dynamic nature of human language. LLMs, on the other hand, have overcome these limitations by being trained on vast amounts of internet text. This training enables them to comprehend and replicate language patterns, grammar, and even sentiments akin to human conversation.
Enhanced Contextual Understanding
One of the most significant advantages of LLMs is their ability to maintain context throughout a conversation. They remember user inputs, previous questions, and responses, allowing for more coherent and engaging interactions. This continuity in conversation is not just a technical advancement but also enhances the user experience, making it more intuitive and satisfying.
Adaptation to Linguistic Nuances
LLMs demonstrate an impressive ability to understand and adapt to the subtle nuances of human language. They can process synonyms, idiomatic expressions, and colloquialisms, enabling them to handle a variety of user inputs effectively. This adaptability allows for more natural and effortless interactions, as users are no longer restricted to specific keywords or rigid syntax.
Advantages of LLMs in Conversational AI
- Contextual Understanding: LLMs excel at grasping the context of conversations, providing relevant and coherent responses, thus making chatbots more human-like.
- Improved Natural Language Understanding: These models surpass traditional methods by adeptly handling complex queries and different writing styles.
- Language Flexibility: LLMs can seamlessly handle multiple languages, a substantial advantage for creating chatbots for diverse linguistic backgrounds.
- Continuous Learning: They can be fine-tuned on specific datasets, allowing for ongoing improvement and adaptation to particular domains or user needs.
In summary, LLMs like GPT-3 have become game-changers in conversational AI, offering substantial improvements over traditional methods. Their ability to understand and generate human-like text, maintain context, adapt to linguistic nuances, and continuously learn makes them invaluable tools for creating smarter, more responsive chatbots and virtual assistants.
Challenges and Limitations of LLMs in Conversational AI
While Large Language Models (LLMs) have significantly advanced the field of conversational AI, they are not without challenges and limitations. Addressing these issues is crucial for the responsible development and deployment of LLM-powered conversational AI.
1. Biases in Training Data
One of the critical challenges facing LLMs is the unintentional inheritance of biases from their training data. These biases can manifest in AI-generated responses, leading to stereotyping or discriminatory behavior. Responsible AI development requires a concerted effort to identify and minimize these biases, ensuring fair and unbiased interactions with users.
2. Ethical Concerns
The powerful capabilities of LLMs also bring ethical concerns, particularly their potential misuse in generating misinformation or deep fake content. Such misuse can erode public trust and cause harm. To counteract this, implementing safeguards, content verification mechanisms, and user authentication is essential to prevent malicious use and ensure ethical AI deployment.
3. Generating False or Misleading Information
LLMs can sometimes produce plausible-sounding but factually incorrect responses. This risk necessitates the incorporation of fact-checking mechanisms and leveraging external data sources to validate the accuracy of AI-generated information, ensuring the reliability and trustworthiness of conversational AI systems.
4. Contextual Understanding Limitations
While LLMs excel in understanding context, they can struggle with ambiguous or poorly phrased queries, leading to irrelevant responses. Continual refinement of the model’s training data and fine-tuning its abilities are vital to enhance contextual comprehension and improve overall user satisfaction.
Addressing these challenges requires a multi-faceted approach, including technological improvements, ethical considerations, and continuous refinement of AI models. By acknowledging and working on these limitations, the conversational AI field can continue to evolve responsibly, ensuring beneficial and reliable interactions between humans and AI.
Newsletter
You Do Not Want to Miss Out!
Step into the Future of Model Deployment. Join Us and Stay Ahead of the Curve!